9 Digital Health Cohorts & Trials
9.1 The emergence of digital studies
We concluded the previous chapter by noting that wearable devices are becoming more widespread and are increasingly able to deliver data that meets the quality standards expected from clinical measurements. While this development has implications for digital public health surveillance, its impact extends beyond that. In Chapter 3, we explored various study types that can provide epidemiological insights, such as cohort studies and randomized controlled trials. Like public health surveillance, epidemiological studies are strongly influenced by the tools and methods of the digital age. Digital cohorts and trials have emerged more recently compared to digital public health surveillance. The earlier development of digital public health surveillance can be attributed to its greater flexibility for experimentation. In contrast, cohort studies and trials tend to be very resource-intensive endeavors, even in their pilot stages, which limits the opportunities for such exploratory approaches. The emergence of digital cohorts and trials in recent years largely reflects the data quality from modern mobile and wearable devices, along with other factors such as widespread adoption of smartphones and internet usage.
Cohort studies and trials are resource-intensive for several reasons, but the primary factor is the frequent interaction between individual participants and health experts for purposes such as recruitment, consent, retention, or data collection. Most of the time, participants must travel to the location of the health experts, such as a study site or a hospital, which incurs time and monetary costs. These inefficiencies contribute to generally low cohort and trial participation rates. Even for diseases like cancer, participation rates are often less than 10% (Unger et al. 2019), with the main factors being trial availability, eligibility, and patient and physician attitudes toward trial participation. Similarly, in recent decades, participation in long-term cohorts has dropped from 80% to 30-40% (Nohr and Liew 2018). Additionally, minorities are often underrepresented in studies, even when they are disproportionately affected by the focal illness of the study (Editors 2021). Digital technologies have the potential to address some of these issues, at least in part. Consequently, there has been a growing interest in how digital cohorts and digital trials can improve study access, engagement, and cost-effectiveness, among other factors (Inan et al. 2020).
It’s worth clarifying what we mean by digital cohorts and digital trials. Building upon an earlier definition of digital epidemiology, I propose defining cohort and trial studies as those enabled by the tools and data sources of the digital age – an era characterized by the widespread presence of computational technology. Such tools and data sources have been a part of clinical studies for more than two decades now. A 2020 study showed that the use of so-called “connected digital products” (e.g., products that capture physiological and behavioral metrics) has grown substantially since 2000, with an annual growth rate of over 30% when including all complete data until 2017 (Marra et al. 2020). In the latter years, the most common types of products were smartphone-enabled technology and mobile apps, reflecting their widespread use.
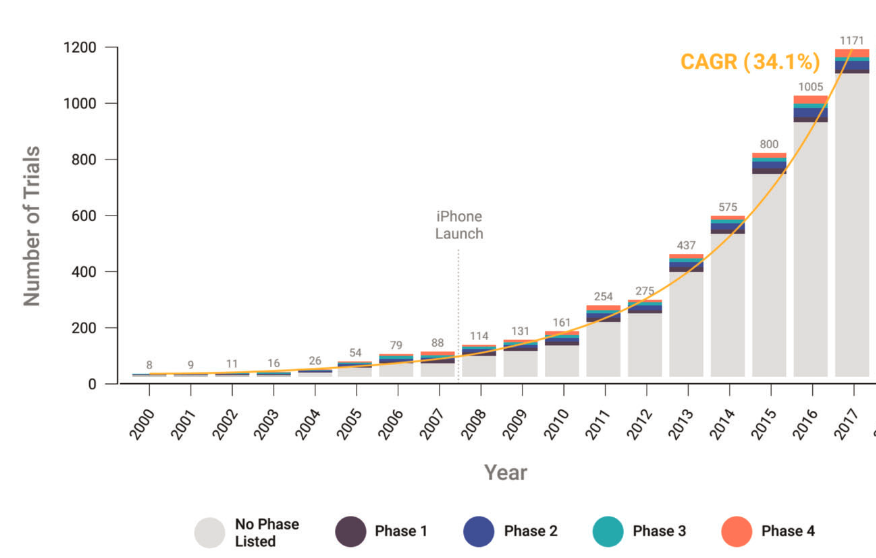
Digital studies are not only characterized by including digital data collection methods but also by using digital technology for recruitment, consent, and engagement. This means that study participants may never have to interact physically with the study team or healthcare professionals, which is why they are sometimes called siteless or decentralized studies (but note that decentralized studies could, in principle, be non-digital if participants have to do site visits). There is thus a continuum of study types reflecting the extent to which digital technology is used. Defining a threshold of digital technology use at which a study can be called a digital study will hardly be helpful. What is clear is that the use of digital technology in cohort and trial studies will only increase, and we will increasingly see studies that are entirely, or almost entirely, run on digital infrastructure.
The terms digital cohort and digital trial are slowly starting to become more commonly used. On the one hand, the concept is relatively new, and the establishment and broad acceptance of terminology takes time. On the other hand, as we’ve seen above, such studies have been referred to as siteless or decentralized studies. Other studies have been called mobile-phone-based studies, mobile health studies, virtual studies, contactless studies, remote studies, and more terms exist. In my view, the terms digital cohort and digital trial integrate well into the overall theme of digital health and digital epidemiology. But perhaps more importantly, the term “digital” captures the main cause of this development. Studies can now be siteless because they are digital, and not the other way around. Mobile health, or mHealth, is an established term but has rapidly been incorporated by the broader umbrella term digital health. For example, the US Food & Drug Administration (FDA) defines digital health to include categories “such as mobile health (mHealth), health information technology (IT), wearable devices, telehealth and telemedicine, and personalized medicine.” Ultimately, which term will become the standard in the long run remains to be seen, and you should be aware that there are currently multiple terms that all refer to essentially the same development.
9.2 Examples of digital cohorts & trials
In the following, we are going to highlight a few studies that can serve as instructive examples of digital cohorts and trials. Recall that the key difference between cohorts and trials is that the former is observational in nature, while the latter is experimental and involves an intervention.
In late 2017, Apple and its collaborators launched the Apple Heart Study to identify irregular heart rhythms, specifically atrial fibrillation1. The study was open to participants in the US who had not previously been diagnosed with atrial fibrillation and owned an Apple Watch. Participation was contingent on providing electronically signed informed consent. Once the watch identified possible atrial fibrillation, participants would receive a free telemedicine consultation, after which they would be sent an electrocardiogram (ECG) patch for further monitoring. After returning the ECG patches by mail, they were interpreted by clinicians. The study found that even though the probability of a participant being notified of potential atrial fibrillation was low (0.52% of 419,297 enrolled participants), among those who were notified, more than a third (34%) had atrial fibrillation confirmed by the ECG patch (Perez et al. 2019). Of those who wore an ECG patch and received an irregular heart rhythm notification simultaneously, 84% were diagnosed with atrial fibrillation. In other words, the positive predictive value of having atrial fibrillation when receiving a notification is 0.84 (95% CI 0.76 - 0.92).
The Apple Heart Study required participants to download a study-specific app. In late 2019, Apple launched the Apple Research app (US only), which allows people to opt into research studies, serving as a single portal for digital studies coordinated in collaboration with Apple. The three studies launched since then are the Apple Women’s Health Study (Mahalingaiah et al. 2022), the Apple Heart & Movement Study, and the Apple Hearing Study (Neitzel et al. 2022). All studies are conducted in partnership with research institutions. Informed consent is provided directly in the app. Most of the studies involve the completion of occasional surveys and sharing some data collected on the phone or the Apple Watch. Similarly, Google launched a portal for digital health studies called “Google Health Studies.” As of early 2023, the portal features two studies: the Digital Wellbeing Study2 and the Respiratory Health Study3. While the studies are ongoing, the first results are beginning to be published. Data from the Apple Women’s Health Study indicated that attempts to conceive during the first few months of the COVID-19 pandemic showed a declining trend compared to pre-pandemic levels (Fruh et al. 2022). In addition, data from the study enabled the investigation of the role of COVID-19 vaccination on menstrual cycle length (Gibson et al. 2022). The study found that vaccinated women experienced a small, non-persistent increase in cycle length for approximately 1-2 cycles post-vaccination. The ability to conduct such pragmatic investigations is a strength of longitudinal cohorts in general, but the digital nature of the studies additionally provides speed and scale.
Whether the model of a portal app will become commonplace remains to be seen. Neither portal has so far seen massive traction, with just five studies launched within three years. Whether the COVID-19 pandemic played a role in this slow start is unclear. Importantly, digital cohorts need not necessarily go through such portal apps. The Apple framework ResearchKit allows researchers and developers to create apps for health research, and numerous digital cohorts have made use of this framework. For example, the MyHeart Counts cardiovascular health study launched in 2015 collected data from over 40,000 participants through a study-specific smartphone app (McConnell et al. 2017). The data included physical activity, health surveys, and sensor readings from a 6-minute walk test. While primarily a feasibility study, results indicated that there was no association between participants’ perception of their activity and sensor-estimated activity, or between their perceived and calculated cardiovascular risk. Similarly, the mPower study was launched in early 2015, collecting smartphone-based sensor data from participants while they performed assessments (speeded tapping, gait/balance, phonation or memory) used to evaluate motor symptoms of Parkinson’s Disease (Bot et al. 2016). The study found that performance on tasks was predictive of self-reported Parkinson’s Disease status and correlated with in-clinic evaluation of disease severity (Omberg et al. 2022). Another study using ResearchKit, the Asthma Mobile Health Study (Chan et al. 2017), collected longitudinal data using a study-specific app. The app collected electronic asthma diary data, tracking asthma symptoms and potential triggers as well as geographically specific weather and pollution information, using phone location services. Increased reporting of asthma symptoms was associated with regions affected by heat, pollen, and wildfires. Google does not currently offer an equivalent framework, but third-party frameworks like ResearchStack4 aim to make apps using ResearchKit easily portable to Android.
The widespread adoption of smartphones has led to a rising popularity of health apps that were not specifically designed for use in cohort studies. However, such apps may provide fertile ground for retrospective digital cohort studies, where the data is used to discover patterns and associations with outcomes of interest. Menstrual tracking apps are a great use case. Such apps, designed to assist women in tracking their menstrual cycles, can provide data to improve our understanding of menstrual health and its connection to women’s health on a large scale. A 2019 study using retrospective self-observation data from two mobile apps – a dataset of more than 30 million days of observations from over 2.7 million cycles for about 200,000 users – found that “follicular phase average duration and range were larger than previously reported, with only 24% of ovulations occurring at cycle days 14 to 15, while the luteal phase duration and range were in line with previous reports, although short luteal phases (10 days or less) were more frequently observed (in up to 20% of cycles)” (Symul et al. 2019). It’s difficult to imagine such population-level insights from self-reported observations without using a digital approach. A 2021 study on an even larger scale, using data from 3.3 million women from 109 countries, found that the menstrual cycle had a greater effect on most measured dimensions of mood (i.e., happy or sad), behavior (i.e., sexual activity), and vital signs (i.e., basal body temperature) than daily, weekly, and seasonal cycles (Pierson et al. 2021).
Digital trials are a relatively new development, and there are only a few successful examples. There are numerous references to digital trials in the literature that upon closer inspection are not trials, but rather cohorts or other non-interventional study types. A randomized controlled trial published in 2005 on the effect of the herbal extract kava and valerian on anxiety and insomnia is the first fully digital trial (Jacobs et al. 2005). It randomized 391 participants into three groups: kava and valerian placebo (n = 121), valerian and kava placebo (n = 135), and double placebo (n = 135). Participants received the study compounds via mail. Outcome measures were reported changes from baseline levels of anxiety and insomnia via a study-specific website. While the study established the feasibility of digital trials, neither kava nor valerian had an effect on anxiety or insomnia that was different from the placebo effect. The REMOTE trial, published in 2014, was the first fully digital trial of a new drug under investigation (Orri et al. 2014). Designed as a phase 4 trial, it was based on previous site-based trials of the same drug, which allowed for the comparison of the two approaches. The drug was shipped to participants’ residences via overnight delivery, and participants had to enter data via a mobile feature phone (Nokia 6301) with a custom study app. While attracting thousands of initial study registrations, high drop-out rates during subsequent verification steps brought down the number of randomized patients to just 18. Experiences from the REMOTE trial were nevertheless highly insightful for future digital trials. Another 2014 study reported results from a randomized controlled trial in children with autism spectrum disorder (ASD), with the goal of assessing the effect of omega-3 fatty acids on hyperactivity (Bent et al. 2014). It randomized 57 children into two groups, one of them a placebo group. All data were collected through the internet, and the intervention was delivered via mail. However, the difference in outcome was not statistically significant in this small trial.
The COVID-19 pandemic provided fertile ground for digital trials. In 2020, a randomized trial of hydroxychloroquine - an anti-malaria drug - as postexposure prophylaxis for COVID-19 was conducted as a fully digital trial (Boulware et al. 2020). It managed to recruit and randomize 821 participants who had high-risk household or occupational exposure to a confirmed COVID-19 case into a hydroxychloroquine (n = 414) and a placebo (n = 407) group. Enrollment, consent, and data collection were done via the REDCap system, a browser-based data capture system5. Both hydroxychloroquine and placebo were shipped overnight to participants’ homes. Due to limited access to testing, COVID-19 status was assigned by infectious disease physicians reviewing participants’ reported symptoms. The incidence among participants did not differ significantly between the groups (Figure 9.2).
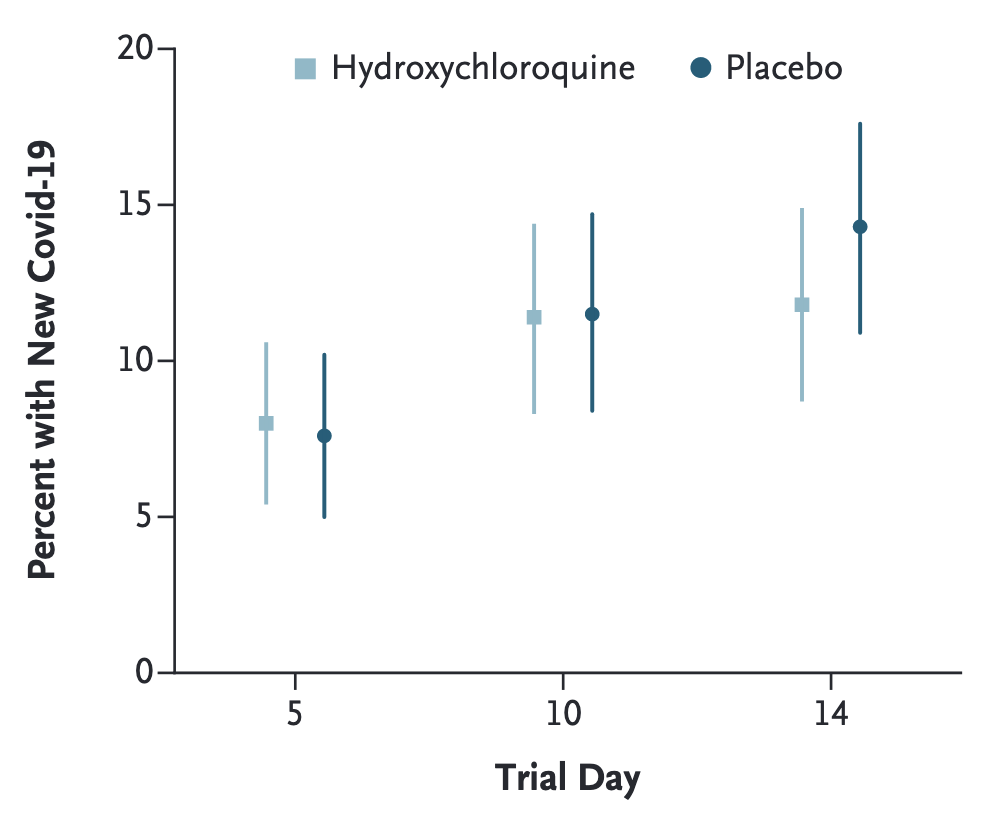
In the same year, another entirely digital randomized controlled trial assessed the effect of fluvoxamine, an antidepressant drug, on clinical deterioration during COVID-19 illness. 152 participants were randomized into a fluvoxamine (n = 80) and a placebo (n = 72) group (Lenze et al. 2020). Participants received both the study medication and various data collection devices, such as an oxygen saturation monitor and an automated blood pressure monitor, delivered to their door. Data collection was done using the REDCap system as well. In this preliminary study, the results indicated that patients treated with fluvoxamine had a lower likelihood of clinical deterioration, but the effect was small.
In 2021, the ADAPTABLE study (Jones et al. 2021) published results from a digital trial, randomizing 15,076 participants into a low-dose (81mg, n = 7,540) and a high-dose (325mg, n=7,536) aspirin group. The outcomes of interest were death from any cause, or hospitalization due to heart attack or stroke, for which the study found no significant difference between the two groups. Interestingly, participant recruitment occurred through electronic health records (EHRs), and data collection was performed through a patient portal website, as well as through EHRs and linkage to private and public health insurance claims data.
9.3 Recruitment, consent, and retention
Every study involving human subjects begins with letting people know that the study exists. This is more difficult than it sounds: participant recruitment is the single biggest cause of clinical trial delays (Ali, Zibert, and Thomsen 2020). A variety of studies looking at incomplete participant recruitment indicate that enrolling a sufficient number of participants is a major problem (Fogel 2018). A study that analyzed initiated phase 2 and 3 intervention trials registered as closed in 2011 found that 19% failed to accrue the necessary number of participants (Carlisle et al. 2015). Given the widespread adoption of the internet today, digital recruitment has the potential of reaching enormous numbers of potential participants. However, getting people’s attention in today’s digital landscape is anything but easy. The Apple Watch study mentioned above had enrolled over 400,000 participants, highlighting that participant access is going to be a key benefit that major platforms offer. Overall, online recruitment is expected to be more efficient than offline recruitment. A 2017 study (Christensen et al. 2017) found that when comparing online and offline recruitment efforts for a digital cohort, more than 80% of participants were recruited with online methods, and the cost for online recruitment per participant was lower.
Once participants are informed about a study and are interested in participating, they need to give informed consent. Informed consent is a key element in any study with human participants, and the idea of providing consent electronically initially caused some concern. However, there is now increasing guidance on electronic consent, for example from the FDA6, which has streamlined the process. In contrast, the situation is more complex in the EU, where there is a large variety of laws and guidelines in the different countries (De Sutter et al. 2022). Informed consent goes beyond simply agreeing to participate in a study - studies must disclose all relevant information, participants must understand what they’re signing up for, and must be able to make a decision free from any influence (Grady et al. 2017). Compared to traditional paper methods, digital methods can dramatically improve this process. A study can provide interactive content to participants, including audio and video. Participants’ understanding of what they agreed to can be assessed with questionnaires that can potentially be personalized, with links to more information. Independent decision-making free from influence is easier because participants can just stop the process without having to say no to a person, which makes many people uncomfortable. Finally, agreement can be gathered in various forms, from checking a box to providing a digital signature. However, while these aspects are certainly positive, there are also downsides. In particular, implementing a digital consent process with all the features described above can be quite complex. Perhaps more importantly, the question of verifying participants - i.e., making sure that they really are who they say they are - can be quite difficult. As most electronic consent guidance documents indicate, verification is not always warranted, but when it is, dropout rates during verification steps can be substantial. A recent review on electronic consent found that the approach is well-received by participants (Skelton et al. 2020). Continued streamlining of the process through platforms like REDCap, Apple Research, or Google Health Studies will further make the process more user-friendly.
Once consented, participants need to stay engaged in the study. This is a further challenge, as a recent review on digital health studies indicated (Pratap et al. 2020). The study analyzed seven digital cohorts and one digital trial, and sought to identify factors associated with increase in participant retention time. Based on data from over 100,000 participants in the US, a survival analysis revealed three factors associated with higher retention rates: older age, having the clinical condition of interest, and being referred by a clinician to the study (Figure 9.3). In addition, while only one of the eight studies compensated participants, that study had by far the highest retention, indicating that monetary incentives could play an important role to enhance engagement. Another review (Daniore, Nittas, and Wyl 2022) looked at 37 digital health studies - defined as longitudinal studies that use mobile technology to perform all key steps online - to assess retention. It tested the hypotheses that incentives and nudges would increase study enrollment and completion, and that increased task complexity would decrease them. However, and perhaps surprisingly, no such effects could be found. One should of course be careful in interpreting these results, as the numbers of digital health cohorts and trials is still relatively small. And while high drop-off rates may be concerning from a study perspective, the data may nevertheless provide key insights into why people stop participating in a study. Future work would certainly benefit from incorporating insights from the social sciences in order to increase enrollment and maximize retention.
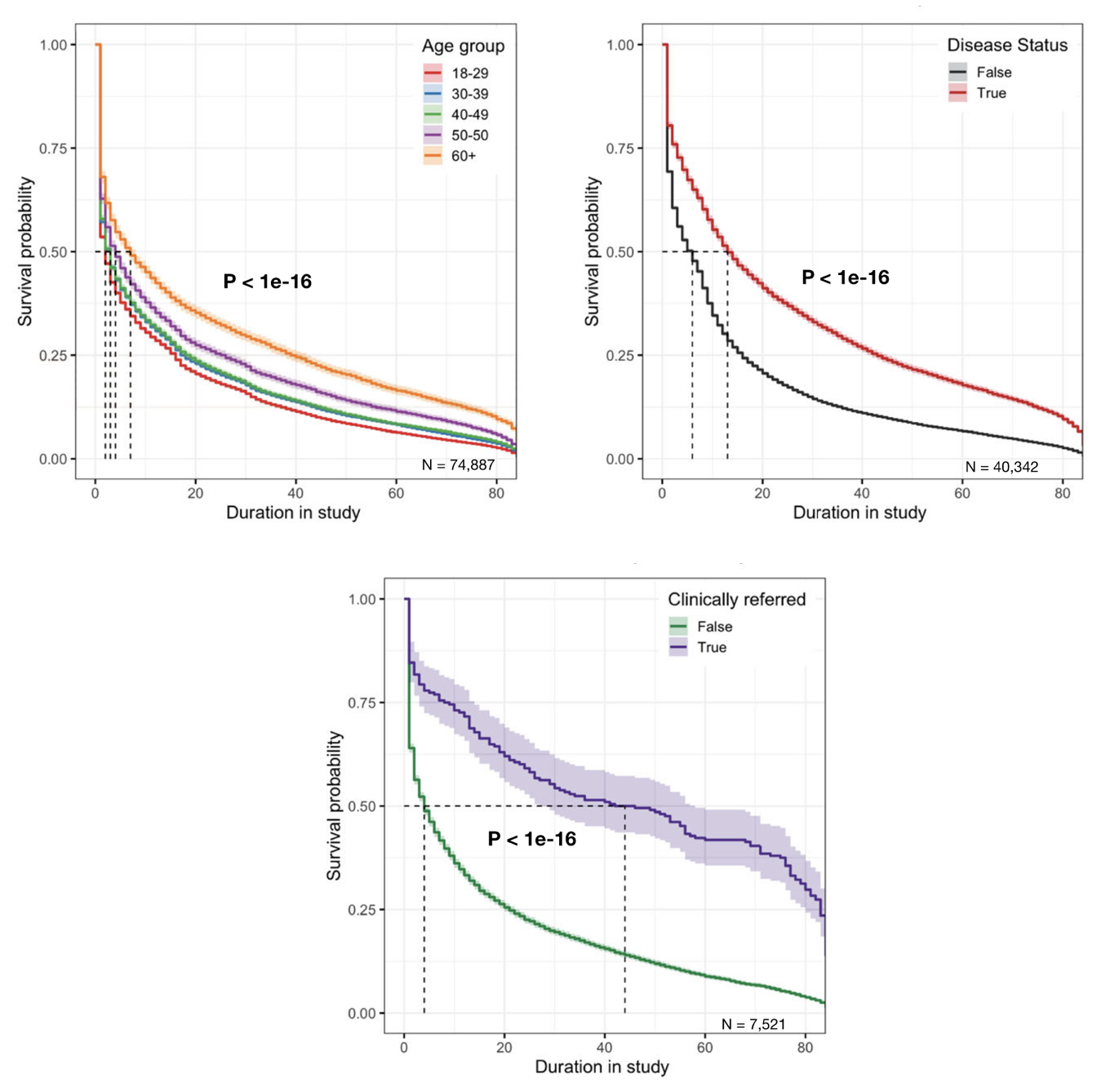
9.4 Data collection
Data collection is at the core of any cohort or trial, and a key aspect about digital cohorts and trials is the digital nature of data collection. Collecting data digitally has a number of important consequences. Perhaps the most important is that data can be collected in the situation in which study participants’ lives unfold, rather than at a clinical site. This in situ data collection - in situ meaning “in the original place” - is a tremendous advantage, because the resulting data has the potential to be a more accurate description of reality than data collected in a clinical environment. Of course, data collected in a clinical environment will continue to be extremely useful, but that is in spite of the location of data collection, not because of it. Clinical data collection benefits from cutting-edge technological and scientific instruments, which can be very expensive, large, and complicated to use. Over time, most technology undergoes a process of miniaturization, and as it evolves for mass usage, it becomes more affordable and easy to use. Furthermore, in situ data collection enables the continuous, longitudinal capture of data, providing a more information-rich picture of the exposures and outcomes of interest. The approach of in situ data collection has a long history in the psychological sciences, where it is known as experience sampling method, or ecological momentary assessment (EMA) (Shiffman, Stone, and Hufford 2008).
In situ data collection means that the participants have to collect the data themselves, which has a number of important consequences. The first is that the work of data collection is handed over to participants, which can have negative effects on retention, as discussed above. A special focus should thus be on the reduction of the burden associated with data collection. In this context, an important difference has to be made between active and passive data collection. Active data collection refers to the collection of data in which participants actively engage in the collection process, for example by filling out surveys. Passive data collection, on the other hand, refers to the collection of data that happens automatically, for example through sensors. As an example, let us take a look at the data collection efforts of the Food & You study7 on personalized nutrition. In this study, participants collected data of various types: demographic data, nutritional data, blood glucose data, physical activity, microbiota data, sleep data, and other data collected by survey. Some of the data was collected actively, including all the survey data, as well as the nutritional data, which participants record though an AI-assisted food tracking app (MyFoodRepo). Some of the data was collected passively, such as the blood glucose levels, which were recorded using an continuous glucose monitor sensor attached to the upper arm. Some data could be collected in either fashion, active or passive, depending on the preference of the participant. For example, some participants have activity trackers or smartwatches, and can thus collect physical activity data passively. Others prefer to report physical activity data in the form of survey responses.
In general, cohorts will increasingly attempt to collect data passively, as the burden on participants is much smaller. Figure 9.4 shows data collection success in the Food & You cohort for blood glucose data, which was collected passively; for diet, which was collected actively; and for sleep and physical activity data, which was collected both actively and passively. The figure clearly shows the higher retention for fully passively collected data. Unless the blood glucose sensor fell off prematurely, the retention is close to 100%. Interestingly, data collection wasn’t fully passive, as participants had to collect the data from the on-body sensor to their mobile phone by hovering their phone over the sensor at least every 8 hours. In cases where the data wasn’t collected after 8 hours, it was lost. This active part of blood glucose data collection led to some data loss, as can be seend in the top panel of Figure 9.4. Similarly, while the percentage of food data is very high (especially considering that participants were asked to track every single dietary intake), there is nevertheless a clear pattern of fatigue visible in the data.
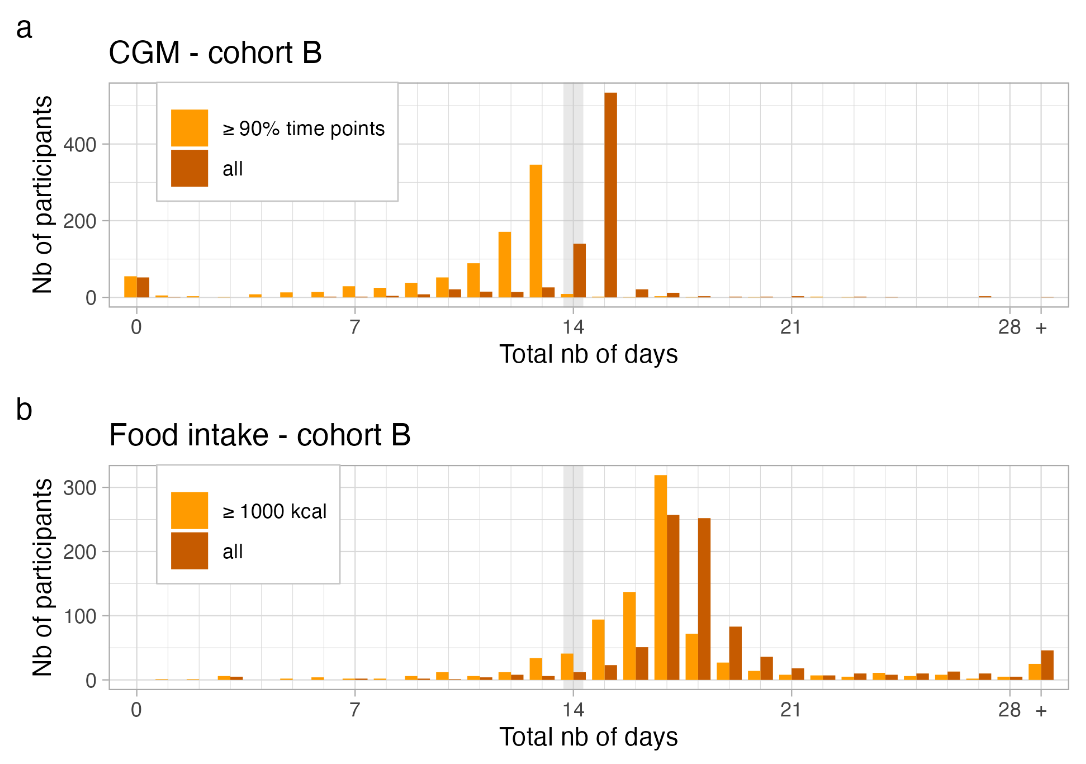
The second important consequence of participant-driven data collection is data quality. Because study investigators are not with the participant when the data are collected, the question about data quality naturally arises. In general, self-reported data is known to suffer from potential biases (Althubaiti 2016), such as the social desirability bias, where participants may preferably provide data that they perceive to be viewed positively by others (a known problem for example in nutrition tracking (Hebert et al. 1995)), or recall bias, where participants don’t remember a particular activity correctly. Objective measurements, such as those coming from sensors, are not free from data quality issues either. These digital biomarkers (Coravos, Khozin, and Mandl 2019) have to be continuously assessed for accuracy and precision. This type of validation, however, typically falls on the shoulders of the producer, rather than on the study investigators, although the validation of a digital biomarker could very well be the focus of a study. In addition, study investigators may even develop bespoke technology to measure an exposure or outcome of interest, in which case they have to ensure themselves that the data they collect are accurate.
As an example, consider the MyFoodRepo app, a mobile app for nutritional tracking, originally specifically designed for the Food & You cohort on personalized nutrition. The app captures nutrition by asking users to take pictures of food, or scan barcodes of packaged products (with a simple text-based fall back option). How much can we trust the data? There are multiple options to increase confidence in the data. First, by the design, the images are assessed by an AI model (Mohanty et al. 2022), and then verified or corrected by a human annotator. Thus, all the data that’s collected is ultimately undergoing manual check. However, it’s still possible that participants forget to collect data, or submit data that does not reflect their actual food intake (for example due to social desirability). The Food & You study team decided to compare the data to two other data sources. On the one hand, a comparison with dietary data previously collected with the help of dietician-assisted 24 hour recalls in the same country (Krieger et al. 2018) showed that the population level dietary statistics, even where stratified by age or gender, were very similar. On the other hand, because participants also wore a continuous glucose monitor, study investigators were able to compare time-stamped dietary data to objective, time-stamped glucose response data. While neither method is perfect, they can provide additional insights into the quality of the data. Further validation studies would shed even more light on the ability of the app to capture dietary intake accurately. Given the rapid development of technology, it’s likely that digital cohorts or trials will want to develop or use bespoke technology to measure exposures or outcomes, which then have to be validated separately. Using such technology early on, or even developing it for a given study, does carry a risk of data quality concerns. On the other hand, waiting until technology is independently validated and widespread carries the risk of missing out on new and potentially much better data sources. Ultimately, it will be up to study investigators to strike the right balance. While exploratory studies may be more open to use new technologies, clinical trials of pharmaceutical interventions will likely be much more conservative in their approach.
9.5 Data analysis
Digital cohorts and trials have the potential to collect an unprecedented variety and volume of data, which presents unique challenges and opportunities in the realm of data analysis. The diversity of data generated through active and passive data collection methods necessitates the development and adoption of advanced analytical techniques to accurately interpret and derive meaningful insights. Multimodal AI (Acosta et al. 2022), which involves the integration of multiple data types and sources, has emerged as a promising approach to address this need.
In digital cohorts and trials, data can be collected through various modalities, such as textual (e.g., surveys, questionnaires), visual (e.g., images, videos), auditory (e.g., voice recordings), and sensor-based (e.g., wearables, continuous glucose monitors) inputs. Each modality provides unique insights into the participants’ behaviors, exposures, and outcomes. However, analyzing these diverse data types in isolation may lead to incomplete or even erroneous conclusions. For example, in the absence of dietary intake data, data on physical activity and blood glucose may easily be misinterpreted as being causal, when the main driver of glucose peaks was the consumed food, which is missing in the data.
Integrating and analyzing multimodal data can be challenging. Each data source has its specific issues, which need to be approached with domain-specific know how. Data for certain modalities may be missing for certain time periods, and may need to be imputed (Smeden et al. 2021). Furthermore, data may be highly distributed for privacy reasons, and thus require decentralized or federated learning approaches to analyze the data while preserving privacy. Additionally, the data may be noisy or unstructured, necessitating the use of advanced machine learning techniques, such as deep learning, to process and extract meaningful patterns. As always, it will be crucial to account for potential biases, both in the data collection process and in the analysis, to ensure valid and generalizable conclusions. Ultimately, the integration of multimodal data necessitates collaboration between domain experts, data scientists, and engineers to develop tailored analytical approaches and models that can effectively handle the complexities and challenges associated with diverse data types coming from digital cohorts and trials.
9.6 The future of digital cohorts and trials
In the previous chapter, we have looked at modern digital participatory surveillance studies such as the COVID Symptom study, and the DETECT study which combined self-reported symptoms with wearable data. There is a strong overlap between such surveillance studies, and digital cohort studies. Such an overlap is not unique to the digital realm: cohort studies have throughout their existence served as a data source for public health surveillance (see also our earlier discussion on public health surveillance as a Swiss army knife). But digital cohort studies go beyond providing data for public health surveillance. As we’ve seen before, one of the key goals of cohort studies is to understand the association of a specific outcome to different levels of exposure. The collection of longitudinal in situ data from digital devices - often referred to as digital phenotyping (Onnela 2021) - allows cohorts to track outcomes and exposures of interest at an unparalleled level of detail. Long-term cohorts, such as the Framingham heart study (initiated in 1948) are now also adopting digital data collection tools. The study launched the electronic Framingham Heart Study (eFHS) app, inviting participants to install the app on their phones while co-deploying a digital blood pressure (BP) cuff and offering a smartwatch (McManus et al. 2019). While these are first steps, the study may one day be fully digital.
As technology advances and data privacy and security concerns continue to evolve, digital cohorts and trials will need to adapt and adopt emerging best practices. Innovations in areas such as federated learning, secure multi-party computation, and differential privacy can help address the challenges of data privacy and security while enabling collaboration and data sharing among researchers. By embracing these advancements and proactively addressing privacy and security concerns, digital cohorts and trials can maximize the potential of diverse data while safeguarding participants’ rights and interests (De Brouwer et al. 2021).
As we’ve seen above, retention is often a key challenge of digital cohorts and trials. Recent developments in artificial intelligence in the form of large language models (LLMs) like GPT have the potential to address the engagement and retention problem often encountered in digital cohorts and trials. With their ability to generate human-like text, LLMs can create personalized and contextually relevant content for participants, enhancing their overall experience. By leveraging these models to tailor messages, reminders, and educational content to individual needs, researchers may soon be able to stimulate motivation and improve adherence to study protocols. Furthermore, LLMs may facilitate real-time, interactive support, answering participants’ questions and addressing concerns promptly, making it easier for them to stay engaged and committed to the study. Indeed, the integration of LLMs into digital cohorts and trials promises to revolutionize the way we approach participant retention, ultimately leading to more robust and representative study results. However, the ethical and legal challenges of integrating bot-driven communication into a digital cohort or trial are not trivial, and we’ll dicsuss some of them in the next chapter.