7 Digital Contact Tracing
While we have mentioned digital aspects of epidemiology in passing before, in this and the following chapters, we will start to look at digital epidemiology approaches in more detail. Before we do that, it might be worthwhile to develop a workable definition of digital epidemiology. We should note at the outset that there is not yet a commonly accepted definition of digital epidemiology (Salathé 2018). For that, the field is still too young.
In the past chapters, we’ve learned many of the key basics of epidemiology. What exactly can digital bring to the field? The shift from mechanical and analogue technology to digital technology has in large parts been brought about by computers. Indeed, computers play a major role in epidemiology. The term computational epidemiology is mostly used in the context of computational models, such as those that we used in Chapter 5 and Chapter 6. This makes sense, because computation refers to the calculation process, which is what these models ultimately represent: sophisticated calculations of mathematical models. When using the term digital, however, I mostly refer to the nature of data, which exists in digital format. It is the digital nature of the data that makes them amenable to further computational analysis.
A first step would thus be to define digital epidemiology as epidemiology with digital data. However, that doesn’t quite sound right - some day, almost all data will be digital, and therefore, with this definition, we would eventually end up with good old epidemiology again, just with digital data. We thus need a better definition. One of the exciting things about digital data is that it is comparatively easy to produce, obtain, and analyze. Before the digital revolution, all epidemiological data had to be collected somewhere, by someone. The digital revolution, with its portable computers, mobile phones, and the internet, has led to a world where millions or even billions of people are producing and sharing digital data that are potentially useful for epidemiology on a daily basis. Consider, for example, social media data. The amount of data that is produced daily is staggering, given that over 5 billion people are now connected to the internet1, many using social media. Some of this data can be used for epidemiological purposes, as we will see later (this is once again a good moment to remind ourselves that we will look into the ethical dimension of all of this as well in a dedicated chapter). With the rise of the mobile internet and social media, researchers began using a lot of data sources in creative ways for the purpose of doing epidemiology, even though the data were never generated for that purpose. Some years ago, this led me to offer a definition of digital epidemiology as “epidemiology that uses data that was generated outside the public health system, i.e. with data that was not generated with the primary purpose of doing epidemiology”(Salathé 2018).
While I still find this definition appealing, the COVID-19 pandemic has brought digital epidemiology to the forefront, with a particular use case that we will discuss in this chapter: digital contact tracing. Digital contact tracing during the pandemic has undoubtedly been the greatest roll-out of digital epidemiology technology in the young history of the field. One would be hard-pressed to argue that digital contact tracing was based on data that was not generated with the primary purpose of doing epidemiology. To begin with, digital contact tracing - at least the privacy-preserving kind that ultimately made it into the operating systems of Apple and Google under the name “Exposure Notification” - hardly produced any data at all. Secondly, and more importantly, digital contact tracing was very much developed with the primary purpose of doing epidemiology in mind.
If I were to offer a more accurate definition, then, it would be the following: digital epidemiology is epidemiology that is enabled by the tools and data sources of the digital age. The digital age refers to our time when computer technology is in widespread use. This definition includes both the digital data sources as well as the tools - such as mobile phones - that generate and utilize much of this data, and it does not ask whether the original purpose of the creation of the data and the tools was doing epidemiology or something else. This definition encompasses both social media analysis as well as digital contact tracing, but excludes the traditional approaches of epidemiology that can be done without any of the tools and data sources of the digital age, or where computers are essentially used by the study team only.
We’ll have to see if this definition stands the test of time, but for the purpose of this book, it is certainly a definition we can work with going forward.
7.1 Conventional contact tracing
Before we get to digital contact tracing, we need to understand in more detail how conventional - i.e. non-digital, or manual - contact tracing works. Contact tracing is a tried and tested methodology in public health. Its ultimate goal is to stop future transmission chains, and thereby help to mitigate an outbreak, or stop it altogether. As we’ve seen earlier, an outbreak continues when infected individuals can pass on the infection to others, who then pass it on to others, and so on. We can use our contact network terminology from earlier to think about the effect of contact tracing. The contacts between individuals - i.e. the edges between the nodes in a contact network - are the substrate (or the roads) on which an infectious agent can move through the network. Using the metaphor of roads, we can say that contact tracing aims to turn nodes into dead ends.
The first step of contact tracing is to identify infected individuals. This is typically done through testing, although symptomatic identification may also be a possibility. Once an infected individual - the index case - has been identified, contact tracing aims to find the individuals who have been in contact with the index case. Here, as elsewhere, the definition of the contact will depend on the transmission mode of the disease in question. Note that we are only interested in contacts that may have been infected by the index case. Thus, there are two conditions for an individual to be the contact of an index case: 1) there must have been a contact with the index case, and 2) the contact must have occurred during the infectious period of the index case. If both conditions are met, then there is a possibility that the index case has passed on the infection to the contact. From the perspective of infection control, when a contact is identified, it is already “too late” for the contact in most cases (but note that there may be post-exposure prophylaxis): the transmission has already occurred, if it has occurred at all. The main goal of contact tracing is to prevent further transmissions from the contacts as the source of the infection by quarantining the contacts (Figure 7.1).
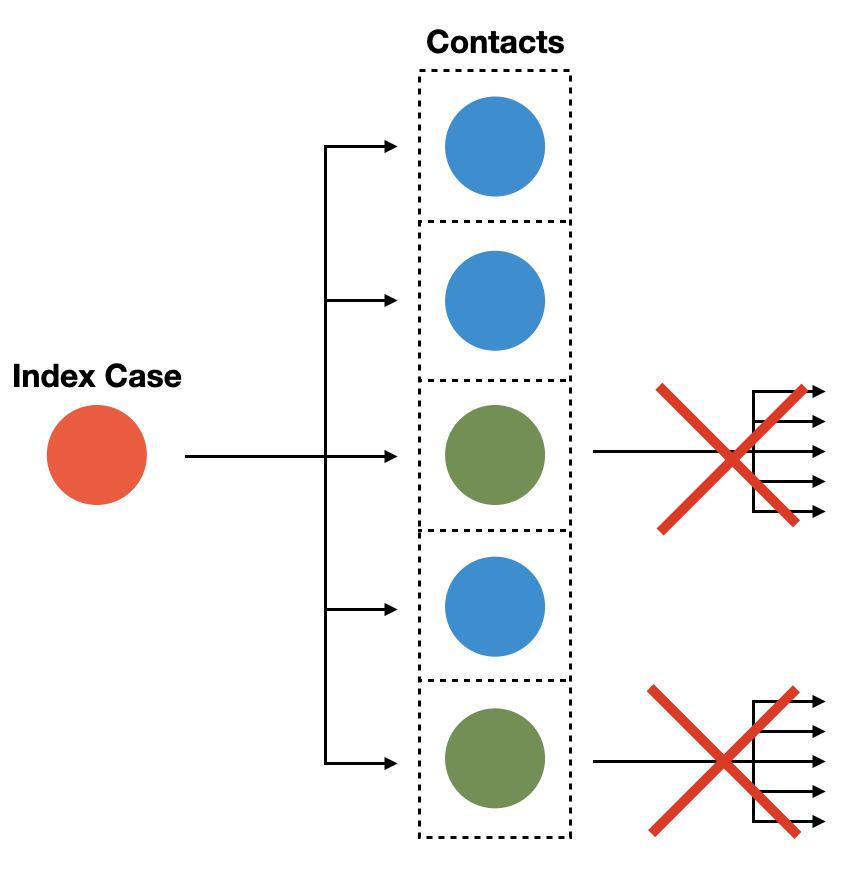
From Figure 7.1, we can also deduce that if we want to prevent all future transmission chains from the infected contacts, we need to adjust the duration of the quarantine according to the incubation period. Note that once an exposed individual in quarantine develops symptoms, or is otherwise confirmed infected (i.e. through a test), the quarantine technically ceases to be a quarantine, and turns into isolation, which will likely go longer than the originally prescribed quarantine. Recall from a previous chapter that the point of quarantine is precautionary isolation, while we don’t know yet whether the exposed contact has been infected or not.
During the COVID-19 pandemic, contact tracing very quickly became a topic at the center of attention. As it was becoming clear that a pandemic was inevitable, contact tracing offered itself as a classical non-pharmaceutical intervention to help and slow down the spread of SARS-CoV-2 (Salathé, Althaus, Neher, et al. 2020). However, much of the conventional contact tracing infrastructure in public health - to the extent that it even existed - had been developed in the context of comparatively slow-moving sexually transmitted diseases (STDs) such as HIV, syphilis, and gonorrhea. In addition, no public health infrastructure was ready for the scale of contact tracing that would be necessary. For example, during the first wave of the pandemic, the Johns Hopkins Center for Health Security published an analysis suggesting that the US would need 100,000 contact tracers, which would cost about 3.6 billion dollars for one year2. Similar suggestions were made in other countries. The world was simply not prepared to leverage this kind of workforce for the task on such short notice.
Contact tracing nonetheless went ahead, with whatever resources were available. A key question at the time was how to define a contact. In early 2020, COVID-19 was assumed to be transmitted either through droplets, or through fomites (we know today that the dominant transmission mode is aerosol, and that fomite transmission is practically irrelevant). Many of the large public health bodies (WHO, US CDC, European CDC, and others) defined a contact as anyone with 1-2 meters for at least 15 minutes. While the time cutoff was essentially arbitrary, the distance was chosen due to limited data suggesting that most large droplets don’t travel further than a few meters. Another key issue was the infectious period. At the time, there was very little data - given the novelty of the pathogen - about the latent period. What seemed to be clear was that there was substantial pre-symptomatic transmission. One of the earliest articles on pre-symptomatic transmission estimated that 44% percent of secondary cases were infected during the index cases’ presymptomatic stage, and that infection occurs 2 to 3 days prior to symptom onset (He et al. 2020). Contact tracing protocols were then adjusted to take this early beginning of the infectious period into account, and contacts were retrospectively traced going back to the beginning of the infectious period (Figure 7.2).
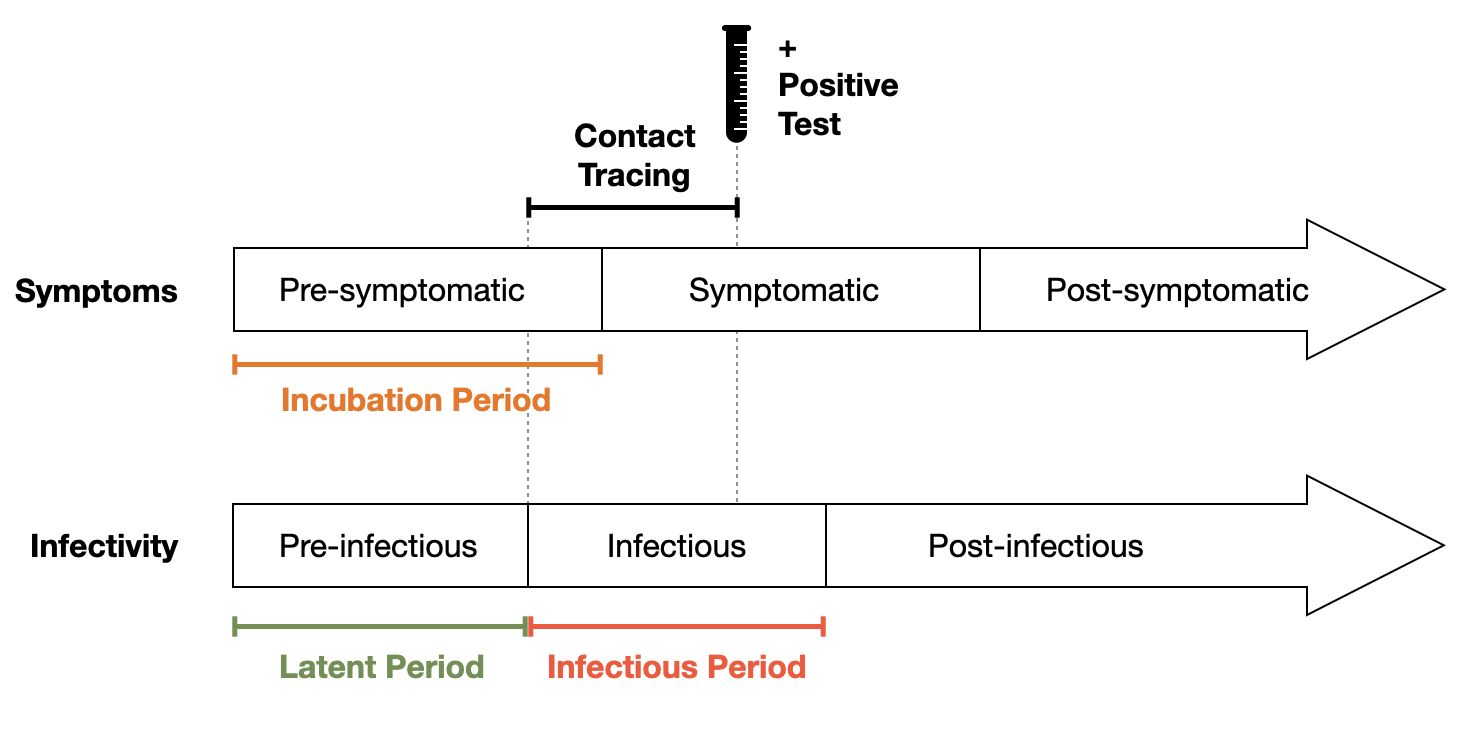
The relatively broad definition of a contact made traditional contact tracing rather difficult. Close proximity interactions could have easily occurred, for example, in public transport, where identifying the relevant contacts would be impossible by people’s memory alone. In addition, contact tracing generally suffers from intrusions into privacy, something that the digital contact protocols tried to tackle as well, as we’ll see below.
A key quantity relevant to contact tracing is the secondary attack rate, or SAR. It is defined as the ratio of infection to exposure, which means that it captures the percentage of people who end up being infected after being exposed to the infection. If contact tracing starting from an index case identifies 10 people having been exposed by the index case, what fraction of those do we expect to have gotten infected? The SAR helps us answer this question. Studies early in the pandemic indicated that the SAR of COVID-19 was in the single percentage digits, and a bit higher (but under 20%) in households (Bi et al. 2020). Over time, as variants became more infectious, household secondary attack rates have increased to over 40% with the Omicron variant that became dominant in late 2021 (Madewell et al. 2022).
It’s not surprising that household attack rates are higher than non-household attack rates, given that household contacts are generally unprotected and long. However, contact tracing should also work outside of households, as household contacts are easy to identify even without the help of contact tracing.
A low SAR also means that many people are in quarantine even though they are not infected. This problem is unavoidable, given that quarantine is precautionary isolation while the infection status is still unknown. However, since vast numbers of people had to go into quarantine during the COVID-19 pandemic, this quickly became an important economical and political issue.
For this reason, there was very strong interest in trying to understand when and under which circumstances people could leave quarantine. In general, the length of the quarantine is determined by the incubation period, as people identified as contacts by contact tracing may have been exposed just very recently. It’s important to note in this context that the variance in observed, individual incubation periods can be large, depending on the disease. For COVID-19, the mean of the incubation period was initially estimated to be around 5-7 days, with observations as long as 14 days or more. Later variants showed markedly reduced incubation periods (Liu et al. 2022). It is illustrative to look at cumulative incubation periods, as shown in Figure 7.3. In the case of incubation periods with a larger variance, as was the case for the earlier variants of SARS-CoV-2, it is reasonable to ask at what point the quarantine should end. To put it bluntly: if a very small number of individuals only start to develop symptoms after 2-3 weeks, is it worthwhile to keep everyone in quarantine for that period of time? Governments around the world offered very different answers to this question.
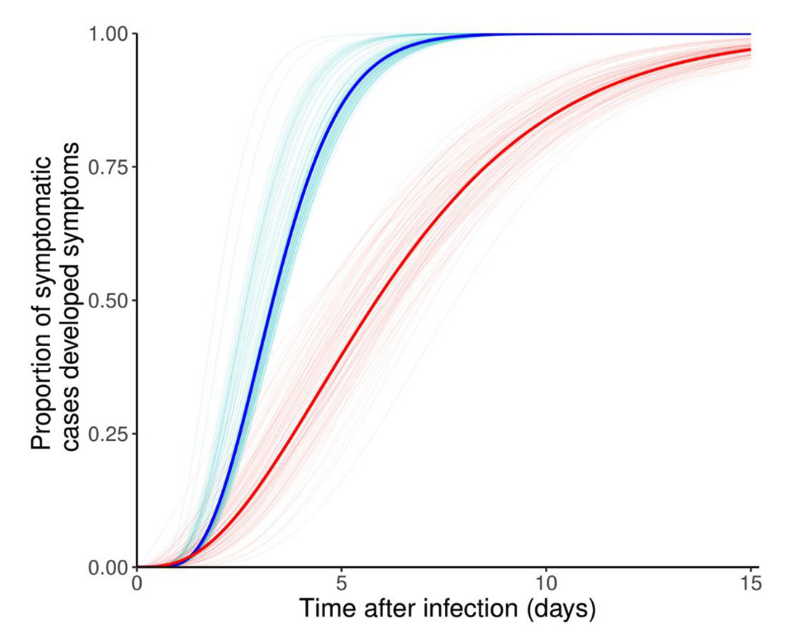
For example, governments who tried to contain SARS-CoV-2 were not willing to run the risk of having some infections slip through the net, as it were, and had rather long quarantine lengths. Governments that were more focused on mitigation were willing to strike a compromise, and minimize quarantine lengths in order to stop most infection chains, but not all. In addition, further quarantine length optimizations were achieved by noting that infections became measurable with RT-PCR tests a few days before the onset of symptoms. Thus, towards the end of the quarantine, “exit testing” strategies were employed to release people from quarantine earlier than a pure symptom-based approach would suggest. Overall, the tradeoff between medical, epidemiological, economical, and social considerations to determine quarantine length was not trivial (Ashcroft et al. 2021).
7.2 Digital contact tracing
The process of TTIQ - testing, contact tracing, isolation, and quarantine - is a tried and tested public health method to mitigate or even contain disease outbreaks. In 2003, it helped contain SARS-CoV-1. A full-blown SARS-CoV-1 pandemic would have been a public health disaster of almost unparalleled proportions, given that SARS-CoV-1 had a mortality of about an order of magnitude higher than SARS-CoV-2. Luckily, SARS-CoV-1 did not transmit during the incubation period, which made effective TTIQ much more feasible.
In the early days of the COVID-19 pandemic, it quickly became clear that conventional contact tracing would be too slow, given the speed at which SARS-CoV-2 spread. Correspondingly, discussions began about how the widespread use of mobile technology could be leveraged to help support TTIQ in the fight against the emerging pandemic. On March 12, 2020, a preprint by Ferretti et al. argued that contact tracing assisted by mobile apps could in principle, through the gain in speed, help contain the pandemic3. At the time, China and other countries already had apps in place to help monitor and manage the pandemic. However, on a global scale, it was clear that personal and legal privacy concerns would not be compatible with many of these early apps.
Digital contact tracing thus faced a dilemma. On the one hand, speeding up contact tracing, and making it more efficient were clearly huge potential advantages. On the other hand, privacy concerns about digital surveillance were substantial, and justifiably so. Contact tracing is already quite intrusive in the traditional version, where individuals have to disclose who they were with, when, and where. This information, when collected centrally and electronically, would quickly offer instant access to abusive population control mechanisms to whoever holds the access keys to the data. Against this background, a number of groups began to develop protocols for digital contact tracing, assigning different importances to these issues. In this chapter, we will not attempt to review all approaches, but will rather focus on the privacy-preserving protocol that was ultimately integrated into the dominant operating systems by Google and Apple (Android and iOS) under the name “Exposure Notification”4, commonly referred to as GAEN.
To understand the use case of digital contact tracing, let us imagine Alice as an average user of a digital contact tracing app (Figure 7.4). She goes about her daily life, and comes into contact with others. At some point, she starts to feel sick, gets tested, and receives a positive test result. At that moment, contact tracing kicks in, with Alice as the index case. Conventional contact tracing begins by a contact tracer contacting Alice, and asking her about her contacts during the previous days, up until 2-3 days before the onset of her symptoms. Alice will try to recall all the contacts she’s had in that time period. She will of course miss some, as she won’t be able to easily identify people she doesn’t know personally. An additional challenge is that she won’t have the contact information of everyone. Once she’s given this information to the contact tracers, they will then inform the contacts about their exposure, and ask them to quarantine.
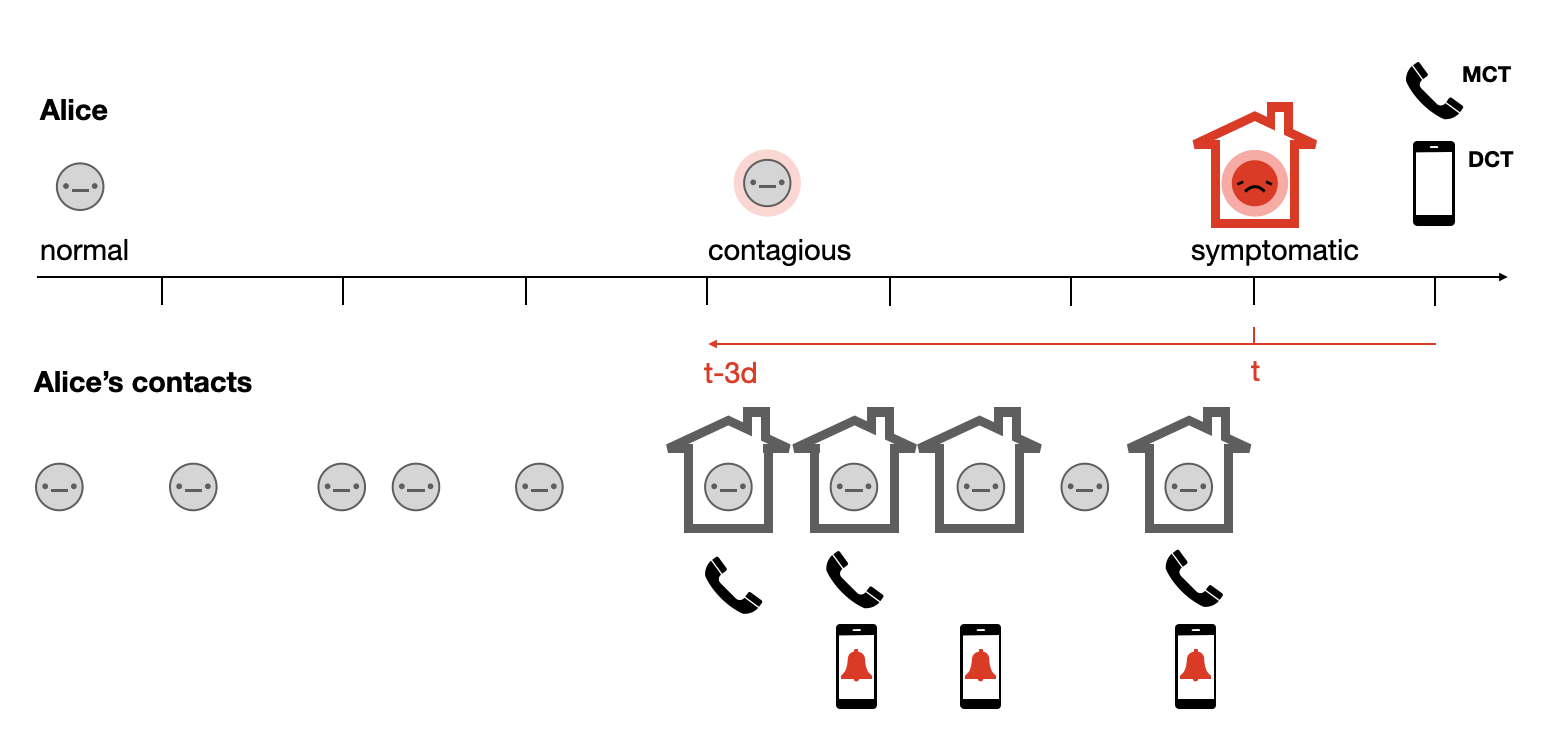
In parallel, digital contact tracing can start if Alice indicates in her contact tracing app that she has been tested positive. Her app may require proof of her positive status - perhaps in the form of an activation code that she received with the positive test result - in order to avoid abuse of the system. Once her app knows that Alice is infected, the digital contact tracing system will notify her contacts who also used the app of their exposure, and ask them to start quarantining. Like the conventional contact tracing approach, the digital contact tracing approach will miss some contacts, either because the contacts were not properly recorded for technical reasons, or because the contact did not use a digital contact tracing app. Her actual contacts may fall into one of four categories: They may either be missed by both approaches; they may be contacted by either digital or traditional contact tracing; or they may be contacted twice, i.e. by both systems. Considering Alice’s example, in Figure 7.4, we can see that she had five contacts during her infectious period before her isolation. Four are reached by the combined contact tracing efforts. If we assume that she passed on the infection to three out of the five contacts, including to the contact who was not reached by either method, the system nevertheless managed to reduce her reproduction number from 3 to 1.
It’s quite intuitive to understand how speed matters in this system. Any delay will mean that the contacts that Alice infected might already become contagious, and pass on the infection, before contact tracing warns them of the exposure. The model developed by Ferretti et al. (2020) showed how instantaneous case isolation and contact quarantine can bring the effective reproduction number below 1, even when a substantial fraction is missed, whereas already a 2 day delay requires almost perfect success in quarantining contacts (Figure 7.5). Quarantining success itself depends on both case and contact using the app, the app correctly identifying the contact, and the contact entering timely and effective quarantine. A tall order for an app, but given what was a stake, this was a path worth exploring.
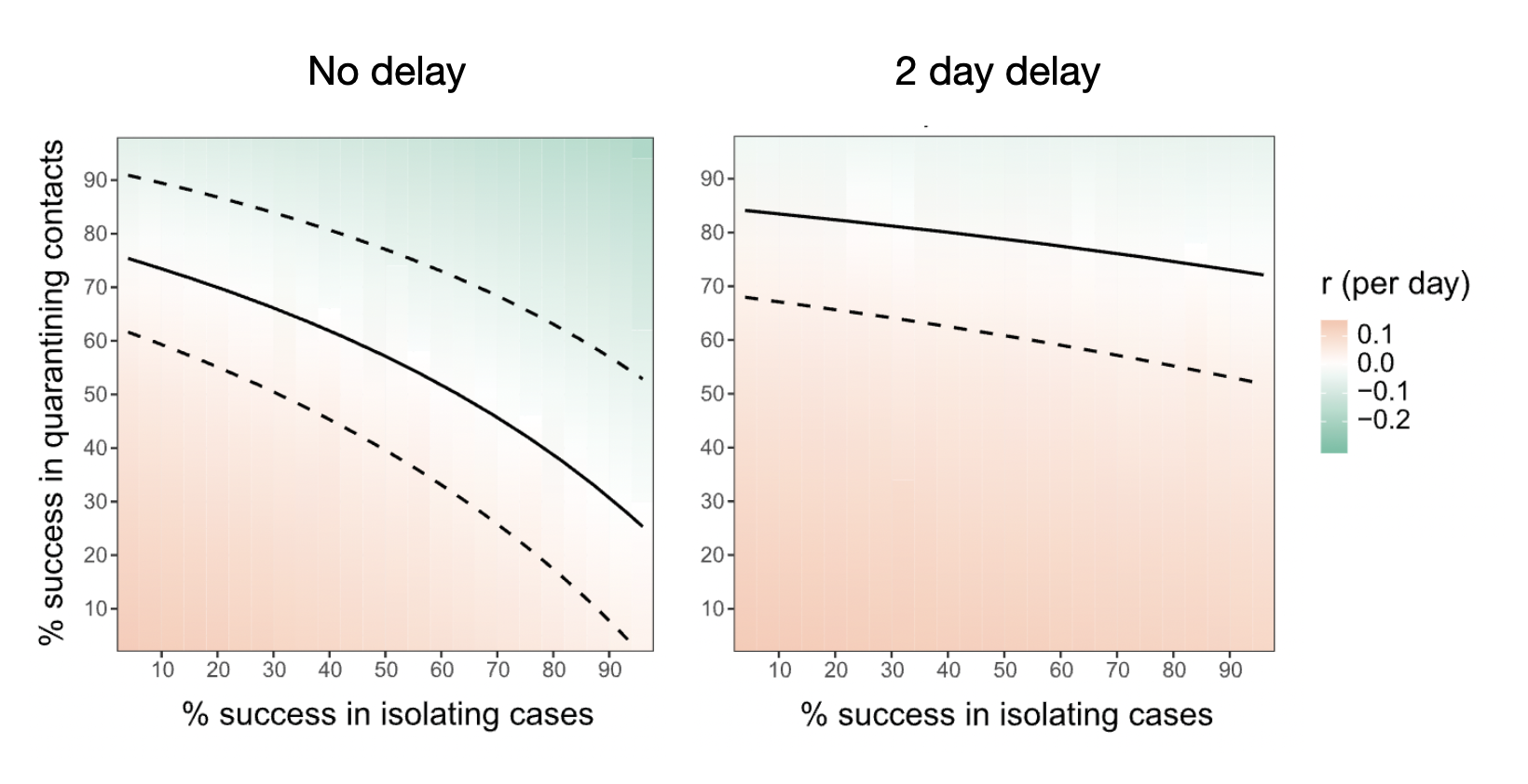
7.3 Privacy-preserving protocols
While the epidemiological benefit of a digital contact tracing app was relatively soon established, and broadly accepted, concerns about the privacy implications quickly became the dominant driver of most discussions. A centralized database of contacts would represent an enormous potential for abuse. At the same time, the attractiveness of using technology to prevent the pandemic was so strong that it led some to suggest that the trade-off should be in favor of pandemic prevention. The following quote from an article in The Economist at the end of February 2020 exemplified this attitude: “People in China, as well as in democracies, worry about how tech companies use the data they garner from their customers. But if covid-19 becomes a pandemic, they may well become more inclined to forgive a more nosy use of personal data if doing so helps defeat the virus.”5. Privacy is important in normal times, the argument went, but in the face of a severe health crisis, those concerns may have to step aside.
In the meanwhile, technologists around the world began to work on privacy-preserving protocols. In what follows, I will explain in more details one of those protocols, the “decentralized, privacy-preserving proximity tracing” protocol, or DP3T (Troncoso et al. 2020). I will put the focus on DP3T because it heavily inspired the development of GAEN (Troncoso et al. 2022) (I should also note that I was part of the team that developed DP3T). DP3T was preceded by an attempt called the “pan-european privacy preserving proximity tracing” protocol, or PEPP-PT, but DP3T split off due to fundamental differences about the technological architecture. Like PEPP-PT, DP3T was also driven by the desire to have a solution that works across political borders. But the key distinguishing feature of DP3T was its decentralized nature, which aimed to prevent all the risks stemming from centralized systems.
In DP3T, a mobile phone continuously generates and broadcasts ephemeral, pseudo-random IDs via Bluetooth Low Energy (BLE). At the same time, the phone also records the pseudo-random IDs from smartphones in close proximity. The proximity itself is estimated using the strength of the recorded BLE signal. While this distance estimate is imprecise, it nevertheless provides a sufficiently accurate assessment of the proximity.
At the time, there was substantial debate in the media about the incapability of BLE signals to obtain an accurate distance measure of 2 meters, which completely ignored that the 2 meters travel distance for droplets was already a highly variable and uncertain estimate. Such “missing the forest for the trees” discussions were relatively common in the development of the technology, which may have contributed to public uncertainty.
Importantly, before any diagnosis of COVID-19, all the data remains on the phone. Upon a positive COVID-19 test, a user can choose to share the information necessary to generate the pseudo-random IDs that were broadcast during the infectious period to a central server. All users of the system regularly download this information from the central server to check locally (on their own phone) if they have been exposed, i.e. in contact with a positively tested person during their infectious period. If that is the case, the app can notify the person of the exposure.
This protocol was called decentralized because, even though a central server was part of the system, all the critical data, and the decision-making, rested decentralized on the phones. This was in contrast to proposed protocols that were centralized, meaning that contact data would be available on the central server, where the decision-making about who to warn of exposure would occur (Figure 7.6).
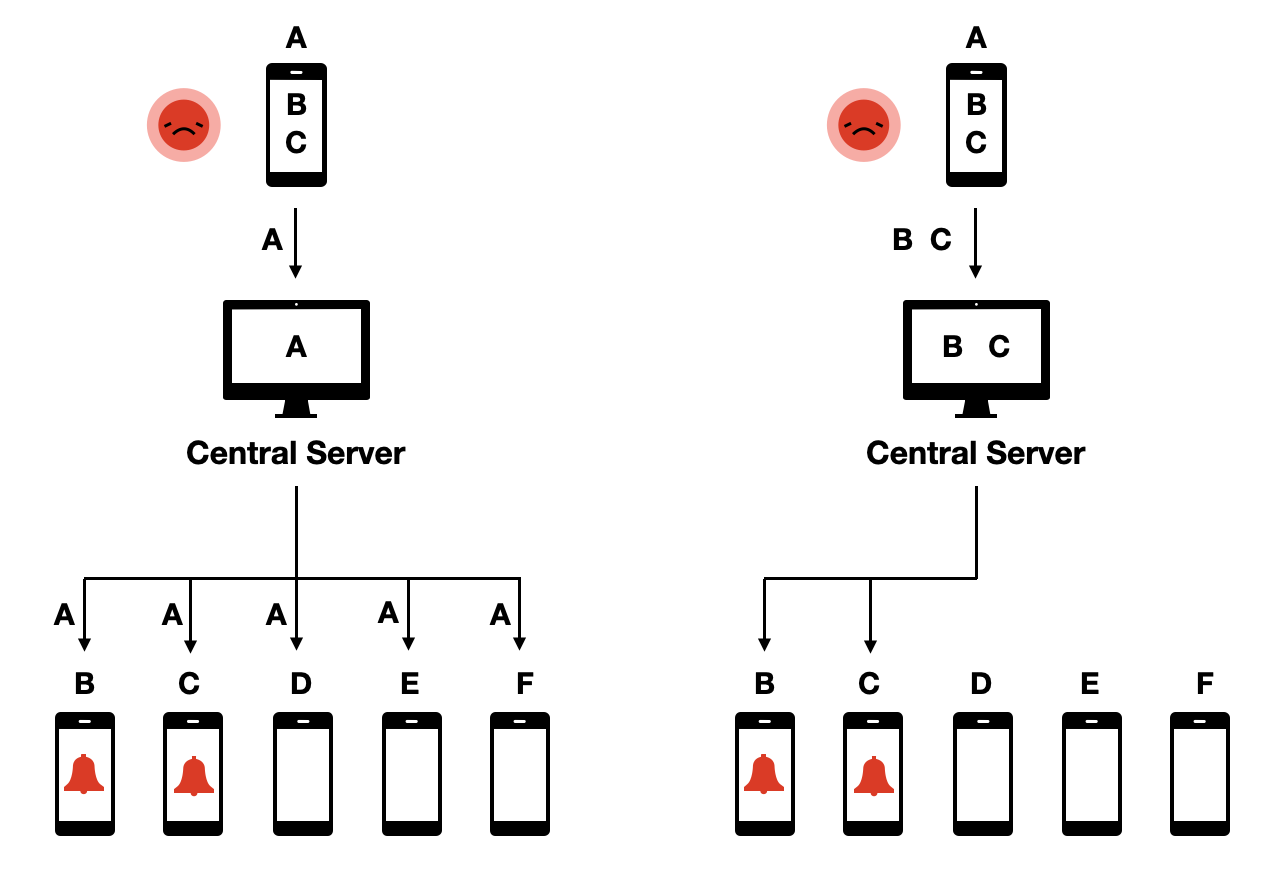
Let us briefly look at the more technical aspects of the DP3T protocol. Upon setup of the contact tracing app, each phone generates a seed \(SK_t\). Each day, the seed is rotated by setting \(SK_{t + 1} = H (SK_t)\), where \(H\) is a hash function.
The phone then generates ephemeral IDs - called \(ephID\)s - using the seed of the day. Each \(ephID\) is valid for a defined period of time, called the epoch. For example, if the epoch is 15 minutes, the phone generates 96 \(ephID\)s daily.
When a phone is in proximity to another phone running the same protocol, it creates a local record of the day, the \(ephID\), and the signal strength with which the data was received.
When a user is tested positive and has received the relevant authorization to activate data sharing, the phone uploads the seed \(SK_{t\_c}\) to the central server, where \(t\_c\) corresponds to the first day when the user has been contagious. After the upload, the phone generates a new seed \(SK_{t}\) to prevent linkability with respect to previous seeds.
In parallel, participating phones periodically download the seeds of infected users. Using the same procedure as described above, the phones reconstruct all broadcast \(ephID\)s, and check whether they match with the \(ephID\)s recorded on the phone. Together with the recorded signal strength, the data can then be used for a local risk calculation, whose result can be turned into a notification of exposure.
The DP3T protocol had a number of properties that made it highly desirable from a privacy point of view. First, it ensured data minimization, given that all sensitive contact data stayed in the hands of app users, rather than being accessible to a central authority. Second, and as a consequence, it minimized the risk of data abuse, because nobody would be able to get access to the data as it was completely distributed decentrally on users’ phones. Third, it made it impossible to track users, because central authorities would not even know that people were using the app unless they voluntarily declared themselves as tested positive. Fourth, it allowed for graceful dismantling - once users decided to stop using the app, the system would stop existing, as no relevant data was stored on a central server that could be leveraged for further contact tracing usage.
The system also has two disadvantages which are important to note. First, because no contact information is transmitted to the central server, the central server cannot directly notify people who have been exposed. Instead, all users must constantly pull the information about infected IDs from the central server, and check locally on their phones if they have been exposed. This adds substantial bandwidth costs to the system. Second, because the central entity has no information about contacts, and their fate with respect to the exposure (i.e. if they later tested positive or not), it is not possible for the system to easily learn in real time more about how to fine-tune parameters to optimize the system. These disadvantages can be circumvented in numerous ways. The bandwidth issue was mostly an efficiency issue, not a critical blocker. Arguably, the fact that the system could not learn more from the collected information was, from an epidemiological perspective, the biggest disadvantage. However, the argument that this wasn’t worth the privacy risk, and that the system could also learn more about the disease with conventual contact tracing, ultimately won out. These kinds of trade-offs are inevitable in digital epidemiology, but understanding all advantages and disadvantages will allow us to find the most optimal solution (depending on how much weight we give to them).
The GAEN protocol follows the decentralized approach. Many health authorities would have preferred the centralized approach, largely out of a desire to collect relevant data and more rapidly understand better the efficacy of contact tracing. Nevertheless, most countries eventually built their apps based on the GAEN protocol. The main reason was that for apps to be effective, they needed to be able to trace contacts even if the phone was not switched on, i.e. in the background. The operating system of iPhones (iOS) however did not allow scanning for Bluetooth signals in the background, mostly for security and efficiency reasons. This functionality was specifically built into GAEN for the purpose of contact tracing only. The implications of operating system providers setting the standards for public health applications continue to be cause for reflection and concern for many public health authorities. We’ll discuss this issue in more detail in the chapter on ethics.
The GAEN protocol is very similar to the DP3T protocol. The seeds are called Temporary Exposure Keys (TEKs) and are generated daily. The \(ephID\)s are called Rolling Proximity Identifiers (RPIs). The generation of RPIs from TEKs is slightly different from the generation of \(ephID\)s from seeds.
7.4 From proximity tracing to presence tracing
Relatively soon after the beginning of the COVID-19 pandemic, it became clear that droplet transmission, which was initially thought to be the dominant transmission mode for the spread of COVID-19, was unable to explain certain patterns, such as the stark difference between indoor spreading (very common) and outdoor spreading (rare), superspreading events, or transmission chains that were known to be over longer distances than those possible with droplet transmission. As the evidence for aerosol transmission began mounting in the summer of 2020, it became clear that digital proximity tracing - as digital contact tracing was called at the time - did not allow to notify people who had potentially been exposed through sharing the same airspace with an infectious person, but were not in close proximity to that person. For this reason, health authorities began to ask for information about presence at a given location, rather than only social contacts, in situations where many people were gathering at the same indoor location, such as workplaces, restaurants, event venues, and others.
Presence tracing refers to the system by which a person can be notified of an exposure at a location at which the person was present. For airborne diseases spread through aerosols, where the infectious agent can linger in the air and travel over longer distances rather than just at close proximity (Wang et al. 2021), presence tracing is very important. Consider the scenario where a SARS-CoV-2 positive person (during their infectious period) enters a room. Virus-laden aerosols will be expelled, and travel to other parts of the room with the help of airflows. A susceptible person could thus be infected at a distance, even though she was never in close proximity to the infectious person (Duval et al. 2022). Furthermore, airborne aerosols don’t evaporate immediately, but can remain in the air for hours. Thus, a susceptible individual may be exposed to virus-laden aerosols expelled by an infectious person who has already left the location. Contact tracing based on proximity would not be able to capture either of these situations.
Figure 7.7 shows the implications from an infectious disease transmission perspective. It considers a simple situation with two rooms, at two different time points. Assuming for simplicity that all individuals are in close proximity to one another in a room, we can map the contact network based on close proximity alone (panel B), or based on a shared airspace (panel C). Note that because of the temporal aspect, the contact network in panel C becomes directional; for example, person 1 being in the room before person 6 means that person 1 could infect person 6, but not the other way around. We can draw two observations from this figure. First, there is substantial overlap between panels B and C, meaning that the exposure notifications that can be triggered from proximity tracing only are still highly valuable. Close proximity transmission remains very important for diseases transmitted by aerosols, because the concentration of aerosols is highest in close proximity to the expelling source, i.e. the infectious person (Chen et al. 2020). Second, there are many new transmission paths that are not captured by proximity considerations only. Indeed, relaxing the assumption in the figure that all interactions in a room are at close proximity would generate even more transmission paths (i.e. those at distance) that would not be captured by proximity alone.
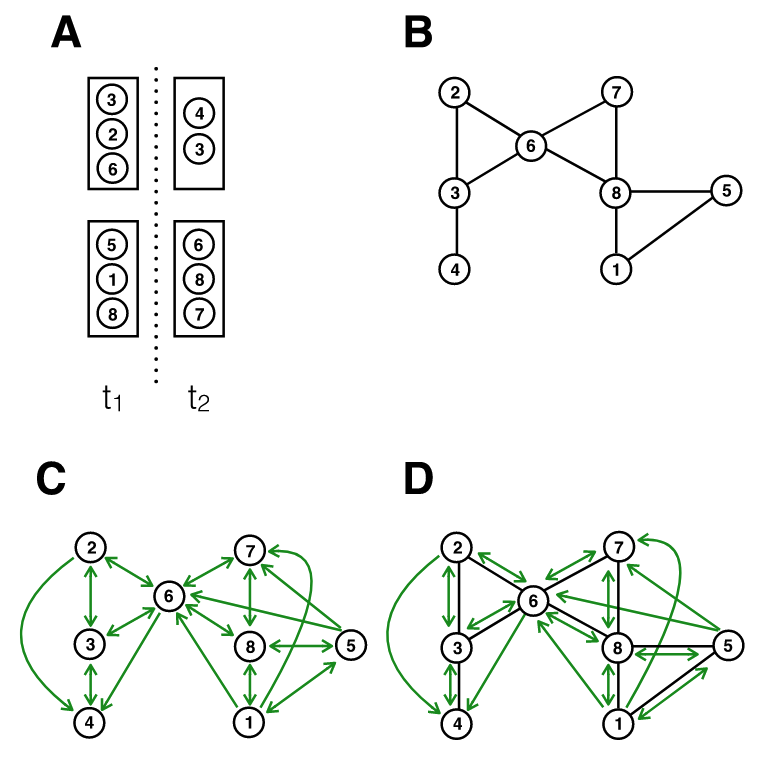
Proximity tracing thus captures only a subset of the exposures that presence tracing would capture. But presence tracing casts quite a wide net - one infected person in a large room could potentially cause hundreds of exposure notifications. To what extent the secondary attack rate would drop by extending proximity tracing to presence tracing is currently not well understood, largely because of the novelty of these systems and the lack of data.
Nonetheless, given the potential importance of SARS-CoV-2 transmission beyond close proximity, health authorities began to collect presence data in various forms. In many situations, paper-based lists were used as a low-tech solution. But relatively quickly, QR code scanning with mobile phones became a dominant mode of data collection. As with proximity tracing, this type of data collection, even though potentially useful for epidemiological purposes, has profound privacy implications. This is particularly true for systems where presence at a given location is recorded electronically and centrally with mobile phones, as it can allow for large-scale surveillance of who was where, and when.
Once again, we can think about protocols that would enable privacy-preserving presence tracing. One such protocol, called CrowdNotifier, was implemented in the German and the Swiss contact tracing apps (CoronaWarnApp6 and SwissCovid7). Similar to proximity tracing, the system is designed to limit abuse by avoiding storing sensitive data centrally, and allows for graceful dismantling. We can again walk through the use case by imagining Alice visiting a venue, such as a restaurant, and scanning the QR code provided by the restaurant. At the same time, Bob, who doesn’t know Alice, also visits the restaurant. Bob sits at another table, and proximity tracing wouldn’t record a contact between them. However, as Bob will find out later, he is SARS-CoV-2 positive and presymptomatically contagious the night of his restaurant visit. Because the restaurant was tightly packed with limited ventilation, the chance of transmission from Bob to Alice was real, and Alice should get notified of the exposure so she could quarantine.
In order to achieve this, the CrowdNotifier protocol requires that the venue owner (let’s call her Carol), produces two types of QR codes; one is a public QR code that she will post in the restaurants for visitors like Alice and Bob to scan; and one is a private QR code that she will use only in the case of having to trigger exposure notifications. Once Bob tests positive for SARS-CoV-2, different options are possible. The system can be designed such that Bob activates the system directly, similar to the proximity tracing system, which prioritizes speed8. It can also be designed to prioritize privacy: once contact tracers learn from Bob that he has been at Carol’s restaurant during his infectious period, they inform Carol that a contagious person was at their place, at which point she scans her private QR code, which triggers a cascade (involving an authorization step by the health authorities to safeguard against malicious use) where the relevant information is made available to all users on a central server. As in proximity tracing, users regularly pull data from the central server to their phones, and their app checks locally - i.e. completely decentralized - whether they have been at a venue that has been contaminated by the presence of a contagious person. No central authority will ever know who else (other than the contagious person) has been at the venue, and yet, everyone who has been there gets notified of the exposure.
Decentralized presence tracing did not become as widespread as decentralized contact tracing, for a number of reasons. First, presence tracing did not require OS updates, and thus the strict approval of Apple and Google. Thus, the diversity of solutions with respect to presence tracing was quite large, and most of these solutions - many coming from private actors - preferred the simplicity and speed of a central system. Second, by the time presence tracing came into focus in the summer and fall of 2020, the pandemic was starting to gain even stronger momentum, as most countries had relaxed measures after the shock of the spring 2020 wave and a relatively calm summer. In the fall of 2020, the public health authorities and the healthcare system struggled under the severe burden of the very high number of cases, and much attention was put on the imminent availability of vaccines, rather than on additional technological development to improve contact tracing. Last but not least, a mix of security concerns and bodged communication in the spring and summer had made contact tracing apps less popular in the general population than initially hoped, and the enthusiasm to continue improving the technology had decreased substantially. We’ll discuss this further below when looking at the future of contact tracing.
7.5 Implementations of digital contact tracing
Digital contact (proximity) tracing was deployed at a speed and scale that was previously unheard of in public health. Some countries began deploying proximity tracing already in March 2020, such as Singapore. However, due to the lack of support for background Bluetooth scanning in iOS (see above), proximity apps at the time didn’t work for a substantial fraction of the population. In April 2020, the two largest mobile OS makers announced a historic partnership on GAEN9. In May 2020, the first pilot apps with the corresponding OS updates for GAEN were deployed. In June 2020, many countries began deploying GAEN-based digital contact tracing at scale. As per Apple’s and Google’s rules on the respective app stores, only one app per country or US state was allowed, and it had to be issued by the corresponding national or state-level health authority. This prevented the creation of a complicated and likely confusing ecosystem of proximity tracing apps.
As we’ve seen above, contact tracing must be integrated into a TTIQ pipeline, i.e. starting with testing and ending with quarantine or isolation. For TTIQ to unleash its full epidemiological potential, all elements in the chain must be effective. Testing must be accurate and easily available, delivering rapid results. Many countries struggled with providing broad access to testing to everyone, and with delivering results quickly. As should be obvious from the timelines on latent periods and infectious periods discussed for SARS-CoV-2, when people have to wait a week to get a test and the result delivered, even the best contact tracing system will be nearly useless. If test results can be obtained rapidly during the course of an infection, contact tracing must be activated quickly. As seen previously, many manual contract tracing systems were overwhelmed by the number of cases. Digital contact tracing systems were in principle easier to activate, but in some instances, the checks built in to prevent abuse of the system, such as activation codes, turned out to be blockers for the rapid activating of contact tracing. For example, the Swiss proximity tracing app SwissCovid required a numerical activation code, which app users were supposed to receive from the local health authorities. However, many codes were delivered with substantial delays, often going beyond five days. This was due to a mix of factors, with the main culprit likely being that the process of generating and delivering activation codes was not automated. Finally, even if exposure notifications arrive in time, will the notified individual go into quarantine? This substantially depends on socio-economic and psychological factors, such as whether the individual is economically incentivized to quarantine, whether people believe that the system works, and others.
Apps around the world tried to address these aspects in different ways, some directly in the app, some in the public health system, and some politically. For example, the NHS Covid-19 app used in the UK allowed users to book a test directly in the app. Test results were delivered to the app, and contact tracing could be activated in this way immediately upon receipt of a positive test result (Wymant et al. (2021)), which substantially accelerated the TTIQ process. However, many public health systems in the world are lagging behind in digitization, and lack the infrastructure to develop, maintain, and secure connected services such as those required by an efficient TTIQ protocol (Troncoso et al. 2022). Further, despite often taking extreme care with respect to privacy considerations, many apps faced a population skeptical of governmental tracing applications (Zhang et al. 2020). Last but not least, early versions of GAEN were not supported on iPhone 6, which in 2020 remained a highly popular model, especially also in the older population (Wyl et al. 2021), an issue that was resolved later in 2021. To further reduce barriers, in 2021, Google and Apple released GAEN Express (“Exposure Notification Express”), an app-less fallback option for users who had GAEN enabled in the OS of the phone, but who had not installed a GAEN-compatible app.
Finally, as envisioned by the early European efforts, which put a lot of cross-border emphasis on the development of the protocol, compatibility of protocols was achieved with the help of a federated gateway system. For example, in Europe, apps from 14 countries in the EU were connected through the European Federated Gateway System10, enabling exchanges between GEAN apps used in those countries. While technically straightforward, the fact that the uploaded data were classified as pseudonymous personal data under GDPR, the deployment of the gateway system was slower than would have been technically possible. In addition, because each country was able to implement its GAEN app with country-specific risk calculations, cross-border agreement of exposure was not trivial, highlighting the need for international standards.
7.6 Effectiveness of digital contact tracing
As digital contact tracing solutions were being deployed on a large scale, the question of effectiveness naturally emerged. Not having been tested in the field before, digital contact tracing was a technology without evidence that it works, even though the model predictions were clear that it should. In addition, there was no a priori reason to assume that the digital version of contact tracing should be any less effective than the manual version. In fact, for all the reasons outlined above with respect to speed, the hope was very much that digital contact tracing would be much more effective than conventional contact tracing.
The decentralized nature of digital contact tracing meant that there was no centralized data that could retrospectively be analyzed. Recall that the central system does not know anything about contacts, or who had received an exposure notification. This was a desired property of the system from a privacy perspective, but it meant that the necessary data for an assessment of efficacy would have to be gathered in another way. In what follows, we will look at three different approaches of GAEN-based apps from Switzerland, Spain, and the UK.
The Swiss study (Salathé, Althaus, Anderegg, et al. 2020) - to the best of my knowledge the first with real-world data - looked at the time period shortly after the launch of the country’s digital contact tracing app “SwissCovid”, from July to September, 2020. COVID-19 cases were relatively low at the time, as the large fall wave hadn’t started yet. In the study time period, 1,645 activation codes were entered into the app, and 16.7% of the Swiss population actively used the app. It is not precisely known how many notifications were triggered by the app, but the system led to 1,695 phone calls to the SwissCovid hotline (calling that hotline was a recommended step by the app after receiving a notification). Most RT-PCR test protocols included a question about whether the subject getting tested had received an exposure notification. During the study period, this number was estimated to be 65 (95% CI 54–77). Thus, the number of positive contacts per index case could be estimated as \(ε = \frac{n}{cμ}\), where \(n = 65\), \(c = 1,645\) and \(μ = 16.7\%\). This formula assumes that contacts of app users are themselves app users with a probability \(μ\), and thus \(ε = 0.24\) (95% CI 0.20 - 0.27), a range similar to numbers reported in conventional contact tracing studies at the time.
An entirely different approach was taken in Spain (Rodrı́guez et al. 2021), which had developed the “RadarCovid” app. To assess effectiveness, a simulation experiment was designed, taking place on an island (La Gomera, one of Spain’s Canary Islands). The local population was invited to participate in an experiment where simulated infections would trigger exposure notifications. Between 29th June and 22nd July 2020, an estimated 33% of the local population of ~10,000 downloaded the app, and four outbreaks were simulated in that time period, where roughly 10% of app users were simulated as infected. Through extensive follow-ups and online surveys, numerous key performance indicators were assessed, including compliance, turnaround time, follow-up, and hidden detection, referring to the percentage of contacts that were strangers to the index case. Overall, the study showed high compliance (64% of users getting an activation code entered it into the app), and detected an average of 6.3 close contacts per simulated infection, about twice the yield from conventional contact tracing. Between 23% and 39% of exposed contacts were strangers to the index case, highlighting a strength of digital contact tracing. The average time between inserting the activation code in the app, and the recommended follow-up call of the contact was 2.35 days, which was slightly faster than the comparable time period reported for conventional contact tracing of 2.6 days. However, only about 10% of the notified contacts followed up by making this call. Whether this low rate was related to the fact that participants were aware of the simulated nature of infections could not be evaluated. Overall, the study suggested that digital contact tracing worked correctly, and provided experimental, even if simulated, evidence for its usefulness, while at the same time highlighting the critical aspect of population support for the method.
The strongest empirical evidence to date for digital contact tracing comes from England and Wales (Wymant et al. 2021). The National Health Service NHS had originally advanced in developing a centralized contact tracing app, but switched to the GAEN protocol in the summer of 2020, and launched the “NHS COVID-19” app on September 24, 2020. Upon installation of the app, users were prompted to enter their postcode district, which allowed for geographical analyses (Figure 7.8). Between app launch and the end of the year, i.e. during the fall wave of 2020, the app was used by approximately 28% of the population. The app triggered approximately 1.7 million exposure notifications - an estimate based on data reported anonymously to the health authorities by the app - which corresponds to 4.2 notified contacts per index case. In comparison, conventional contact tracing triggered 1.8 contact notifications per index case, and thus like in Spain, the yield of digital contact tracing was substantially higher. The secondary attack rate (SAR) was estimated at 6%, which was similar to the SAR for manually traced contacts.
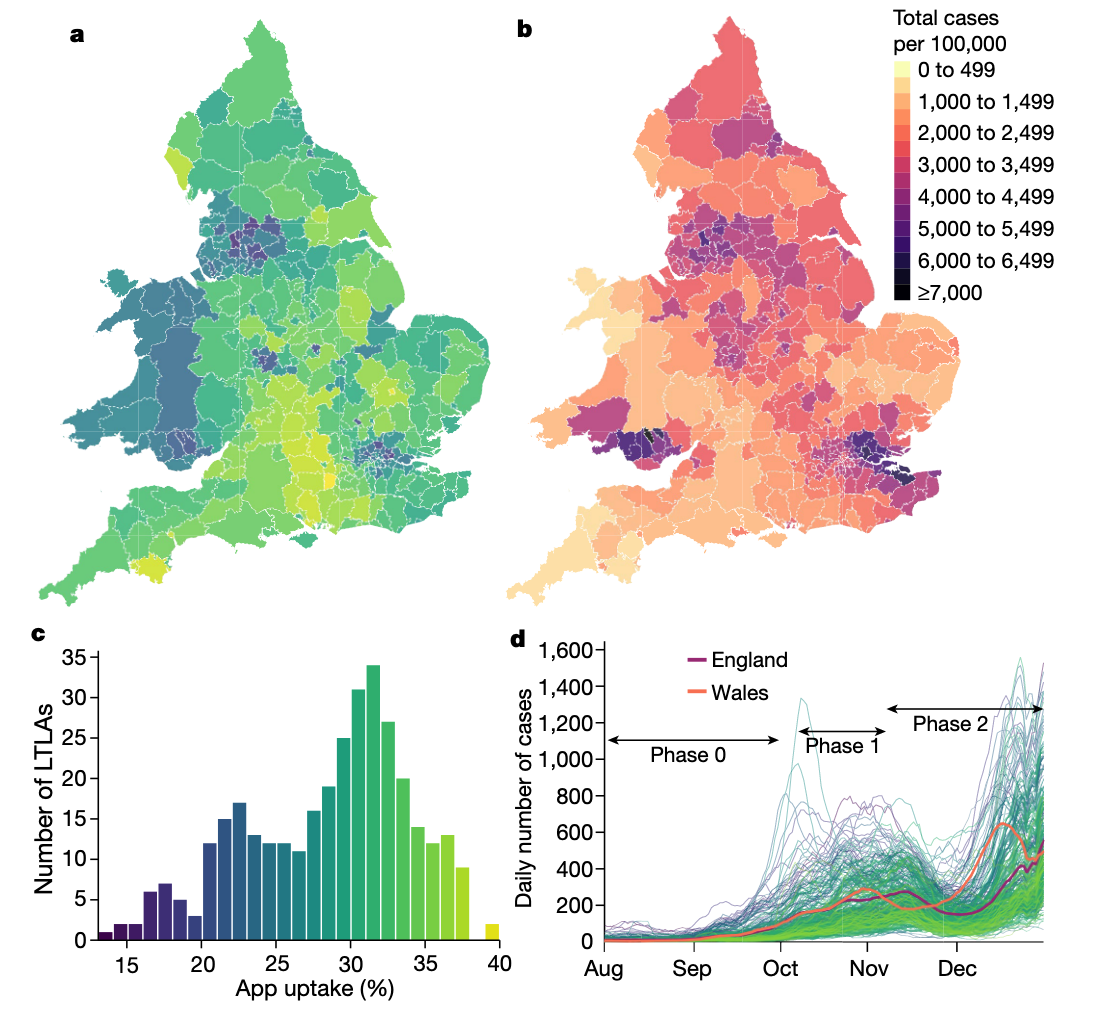
To understand the epidemiological impact of the app, the study used two complementary approaches. The first was to use a modeling approach to understand the effect of a notification, which took into account the number of notifications, the SAR, the expected fraction of transmissions prevented by quarantine, quarantine adherence, and an estimate of the expected size of the transmission chain that would occur in the absence of the notification. The second approach leveraged the fact that app users indicated their postcode district upon app installation. An analysis of the level of local authorities linked geographic variation in app uptake with geographic variation in cumulative case counts, taking into account confounding factors at the geographic level. Both analyses estimated that during the study period of a bit more than three months of the fall 2020 wave, digital contact tracing prevented between 284,000 and 594,000 cases, with the combined 95% sensitivity analyses showing a range of 108,000 to 914,000 cases prevented. Correspondingly, taking fatality rates at the time into account, the estimates for averted deaths due to digital contact tracing were 4,200 to 8,700 (1,600 – 13,500). Further analysis showed that for every percentage point increase in app uptake, the number of cases averted was 0.8% (using the modeling approach) and 2.3% (using the geographical analysis) (Figure 7.9).
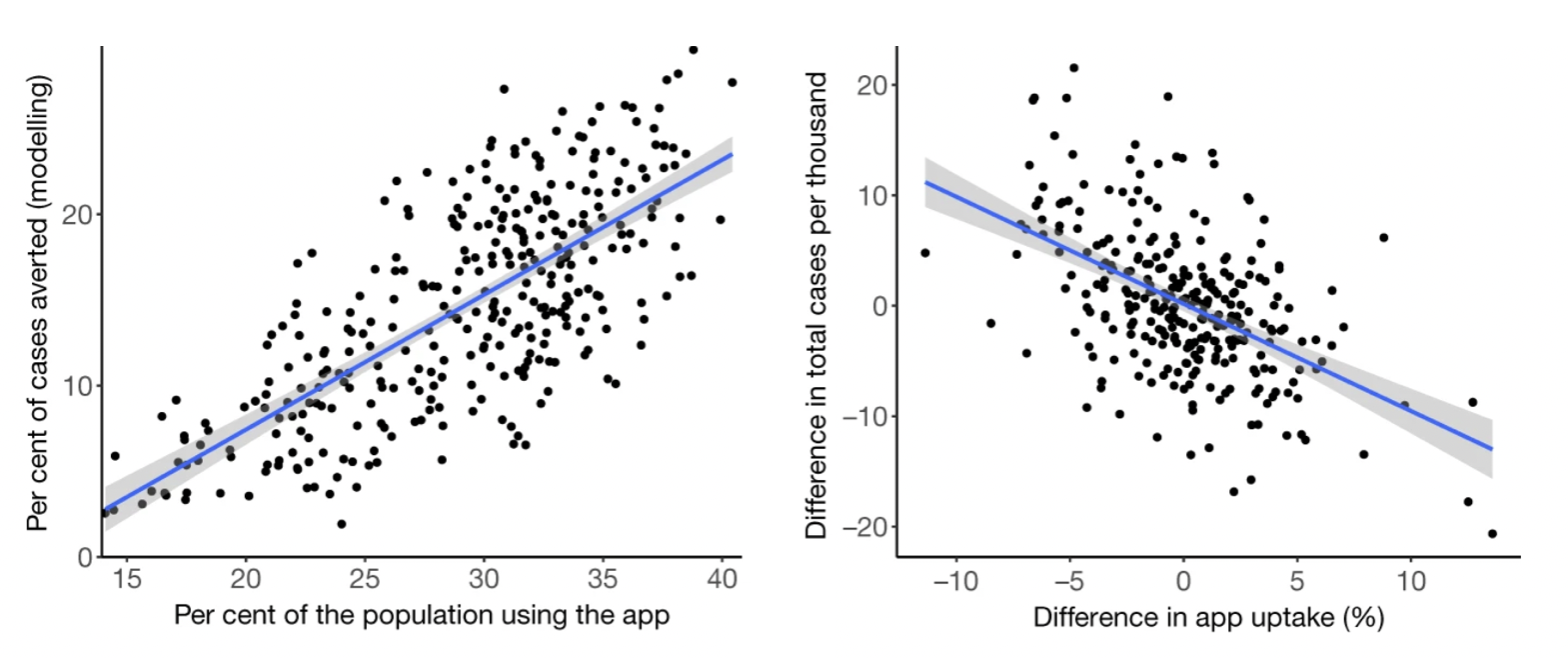
The effectiveness of digital contact tracing has in the meanwhile been further validated through comprehensive longitudinal studies. Kendall et al. (2023) demonstrated that the NHS COVID-19 app averted approximately 1 million SARS-CoV-2 cases during its first year of operation, corresponding to 44,000 hospitalizations and 9,600 deaths. This impact varied according to changing social and epidemic characteristics throughout different waves. The app’s epidemiological impact was especially evident in its ability to identify infectious contacts well beyond manual tracing capabilities. While manual tracing typically reached 1.8 contacts per index case, digital tracing reached 4.2 contacts per case. Furthermore, app-notified individuals were consistently more likely to test positive than the general population, with this likelihood being up to 26 times higher. These findings provide further compelling evidence that privacy-preserving digital contact tracing can effectively complement manual approaches, particularly during periods of high case prevalence when manual systems become overwhelmed.
Taken together, such studies provide emerging evidence that digital contact tracing is an effective addition to the arsenal of tools to fight infectious disease outbreaks. An EU report published in December 2022 summarized some of the then available evidence and concluded that “best practice examples show that the apps can be a powerful tool to complement conventional contact tracing and support public health processes, by consistently identifying contacts that tested positive which would otherwise be missed by conventional contact tracing”11. Given what we learned during Pandemic, especially from the UK rollout, the evidence strongly suggests that decentralized digital contact tracing can fulfill its key promises of providing rapid notifications to relevant contacts, including many that would not be notifiable through conventional contact tracing, all while strongly preserving privacy. In doing so, it can prevent cases and deaths, but its efficacy very much depends on app uptake rates and efficient integration into the TTIQ pipeline. Initial fears about rampant false-positive notifications overwhelming the healthcare system did not materialize.
7.7 The future of digital contact tracing
As vaccines against COVID-19 became widely available, and the threat of high mortality or severe disease began rescinding, most countries eventually stopped their contact tracing and quarantining efforts, and discontinued their digital contact tracing apps. Today, digital contact tracing is still employed in certain parts of the world, but the large-scale deployment has come to an end. Beyond the empirical evidence for its efficacy, what learnings can we draw from the historically biggest roll-out of digital epidemiology technology?
First, the tradeoff between privacy and epidemiological impact is much smaller than generally perceived. Digital epidemiology is often perceived as weak on privacy, but the decentralized tracing protocols demonstrated that the goals of digital epidemiology needn’t be at odds with privacy. Interestingly, in surveys, many people reported not using digital contact tracing apps because of privacy concerns (Wyl et al. 2021; Gao et al. 2022). One can’t expect the general population to always be on top of the privacy-relevant details of contact tracing protocols. Trust in technology is ultimately trust in the provider of the technology. If people don’t trust the providers - from governments to tech companies - then even the best privacy-preserving solutions will be rejected.
Privacy must be built into the technology from the start (privacy by design). Solutions that did not follow this principle - such as centralized approaches - argued that people should put their trust in central authorities to not abuse the data. However, even with the best of intentions, centrally stored data can lead to catastrophic data leaks at scale. In addition, the availability of information-rich data in central places often turns out to be too attractive. For example, despite assurances to the contrary, centralized contact tracing data in Singapore (which opted for a centralized approach) was used by police for criminal investigations on multiple occasions12. Similarly, German police used data from the private, centralized presence tracing app Luca for criminal investigations13 (note that the official government app, CoronaWarnApp, uses decentralized presence tracing). Such abuses of trust will unfortunately hamper widespread adoption of contact tracing apps, even of those that have privacy built in by design.
This leads us directly to the second learning, which is on the importance of good and continued communication. The epidemiological impact of digital contact tracing scales directly with the uptake rate. As the NHS COVID-19 app study showed (Wymant et al. 2021), each additional percentage point would lead to around one percentage point in cases averted. One does not need to use advanced mathematics to understand just how impactful digital contact tracing could be if the vast majority of people used it. But for this to happen, there needs to be clear communication on the trustworthiness of the technology (provided it exists), and continued dialogue about how the technology and the broader TTIQ method work. What might be obvious to a public health professional may not be so clear to the general population.
Digital contact tracing faced particular challenges, as people who are experts in technology often pronounced themselves critically, and without competence, on the epidemiological aspects, and vice versa. As a typical example, take the following excerpt from Buzzfeed14, on April 29, 2020, a hot phase of digital contact tracing technology:
“My problem with contact tracing apps is that they have absolutely no value,” Bruce Schneier, a privacy expert and fellow at the Berkman Klein Center for Internet & Society at Harvard University, told BuzzFeed News. “I’m not even talking about the privacy concerns, I mean the efficacy. Does anybody think this will do something useful? … This is just something governments want to do for the hell of it. To me, it’s just techies doing techie things because they don’t know what else to do.”
If experts in privacy technology dismiss new digital epidemiology approaches out of hand, before it is deployed and before the evidence has been gathered, we should perhaps not be surprised that the general population does not adopt the technology broadly. This is particularly concerning as there is some evidence the perceived lack of benefit has been a main inhibitor of broad uptake (Wyl et al. 2021; Gao et al. 2022). While it’s possible that the complete novelty of digital contact tracing combined with the shock of an unparalleled health crisis may have contributed to the cacophony of reactions, one can only hope that in a future pandemic, people will look at the now available empirical evidence before pronouncing themselves in public.
Once people start using a digital contact tracing app, communication doesn’t end there. The app is itself a major communication vehicle that countries and states have used in different ways, and we need to understand better what worked and what didn’t. The development of digital contact tracing apps was so fast that such considerations were initially not on any priority list. While this is understandable initially when launching an app in response to a new pandemic, communication should have received major attention over the following weeks and months after release. Let me highlight two examples from the Swiss app which are likely representative of problems encountered elsewhere. First, the Swiss app decided early on that the app should primarily be a “background app”, meaning that it would simply do its job silently, and only alert the user if there was something to be concerned about, i.e. an exposure worthy of a notification. In contrast, other apps, such as the German Corona Warn app, were constantly communicating different risk levels to the users, using a color code. As a consequence, many Swiss users over time began to wonder whether the app worked at all, since it wasn’t signaling anything to them. Second, many apps began building additional information streams and functionalities into their contact tracing apps. For example, some apps began to integrate vaccination certificates into the contact tracing app, or dashboards about local disease activity. The Swiss app decided against such additional functionality, as there was concern that such bundling would make it harder to dismantle parts of the system once they were not necessary anymore. Which approach is more useful depends critically on an app’s objectives, but we should be mindful that communication from the app plays a key role in its future use.
The third learning is that based on our current understanding of the dominant transmission mode of SARS-CoV-2 and other respiratory pathogens, it is likely that presence tracing will play a much bigger role than proximity tracing in future pandemics of respiratory diseases. Presence tracing has yet to be validated with empirical evidence, but it’s clear that future digital contact tracing cannot focus exclusively on transmissions over short distances. Presence tracing solutions have often been deployed with privacy as a secondary thought, if at all, and thus a particular focus should be put on developing standards to ensure deployed solutions benefit from the same privacy guarantees as decentralized proximity tracing. The lack of such standards would almost certainly lead to abuses of centralized data (as seen during the COVID-19 pandemic), which would erode trust in all digital contact tracing applications.
Fourth, a more general learning is that many healthcare systems are relying on extremely outdated technology (e.g. fax), or even manual processes. These systems need to rapidly advance their digital capabilities. The TTIQ methodology operates in an environment with many different stakeholders, making technical coordination even more important. Those aspects of the TTIQ pipeline that were not automated often proved to be the least reliable, especially as systems began to experience extreme stress when case numbers were high, giving end users the impression that the digital contact tracing app didn’t work when it would have been most useful.
Fifth, the rapid development of contact tracing technology led to a wide range of terminologies, and approaches to assessing the performance and efficacies of digital contact tracing systems. Common frameworks to evaluate the apps (Colizza et al. 2021), their impact and terminology (Lueks et al. 2021) are urgently needed. Finally, and sixth, there is an urgent need for international standards for risk calculations (i.e. the conditions under which an app should trigger a notification). This will make apps not only more comparable, but it will also make their integration across international borders much easier.
Lastly, we should not forget that digital contact tracing can also teach us certain things about infecitous diseases that would otherwise be hard to understand. For example, an analysis of 7 million exposure notifications from the NHS COVID-19 app in England and Wales showed that the probability of transmission increased initially linearly with duration of exposure at a rate of approximately 1.1% per hour, continuing to increase over several days (Ferretti et al. 2024). This research validated a key principle of contact tracing: longer exposures at greater distances carried similar risk to shorter exposures at closer distances, confirming that both time and space dimensions matter for transmission. While most exposures recorded were brief (median 0.7 hours), transmissions typically resulted from considerably longer exposures (median 6 hours). Analysis showed that 82% of transmissions occurred from exposures lasting more than one hour, suggesting that contact tracing for respiratory diseases like SARS-CoV-2 could maintain most of its effectiveness with a 1-hour threshold rather than the commonly used 15-minute threshold. Additionally, household and recurring contacts, while representing 20% of all app-recorded contacts, were responsible for 65% of transmissions—a disproportionate impact explained by their longer duration and closer proximity. These quantitative risk measurements enable optimization of various management strategies and support the development of more targeted interventions based on simple, effective predictors such as exposure duration. With sufficient preparation, similar privacy-preserving analyses could be performed within weeks of a new pathogen’s emergence, substantially improving the response to future epidemics.
While contact tracing has seen very rapid development during the early phase of the pandemic, its development has come almost to a full stop. This isn’t smart (Salathé 2023). When the next pandemic hits (when, not if), we should not spend valuable time developing new technology under extremely difficult circumstances that could have been developed in quieter times before. As is always the case with infectious diseases, the earlier an intervention can have an effect, the more outsized its impact will be.
https://www.centerforhealthsecurity.org/our-work/publications/2020/a-national-plan-to-enable-comprehensive-covid-19-case-finding-and-contact-tracing-in-the-us↩︎
https://www.medrxiv.org/content/10.1101/2020.03.08.20032946v1?versioned=true↩︎
https://www.google.com/covid19/exposurenotifications/, https://developer.apple.com/exposure-notification/↩︎
https://www.economist.com/china/2020/02/29/to-curb-covid-19-china-is-using-its-high-tech-surveillance-tools↩︎
https://www.coronawarn.app/en/blog/2021-04-21-corona-warn-app-version-2-0/↩︎
https://www.ncsc.admin.ch/ncsc/en/home/dokumentation/covid-public-security-test/infos.html↩︎
https://github.com/corona-warn-app/cwa-documentation/blob/main/event_registration.md↩︎
https://www.apple.com/newsroom/2020/04/apple-and-google-partner-on-covid-19-contact-tracing-technology/↩︎
https://health.ec.europa.eu/publications/european-interoperability-certificate-governance-security-architecture-contact-tracing-and-warning_en↩︎
https://op.europa.eu/en/publication-detail/-/publication/69f8bd22-7065-11ed-9887-01aa75ed71a1↩︎
https://en.wikipedia.org/wiki/TraceTogether#Controversy_over_police_access↩︎
https://www.dw.com/en/german-police-under-fire-for-misuse-of-covid-contact-tracing-app/a-60393597↩︎
https://www.buzzfeednews.com/article/carolinehaskins1/coronavirus-contact-tracing-google-apple↩︎